Friday, November 15, 2019
8:30 a.m.–2:20 p.m.
breakfast (doors open), 8 a.m.
Hiebert Lounge
72 East Concord Street
Boston
Services for Deaf and Hard-of-Hearing People Provided
#StatisticsLifeSciences
Livestreaming Available During Event
Planned in coordination with the Boston University School of Public Health Department of Biostatistics and the Boston University Department of Mathematics and Statistics, and cohosted by the American Statistical Association, the Institute for Mathematical Statistics, and the National Institute of Statistical Sciences.
We are globally connected like never before, in nearly all aspects of our lives. While this fact has numerous implications, from the perspective of public health it leaves us uniquely poised to potentially overcome major challenges that have to date been out of reach. These include aging traits such as cognitive decline and Alzheimer’s disease, pulmonary disease such as COPD and asthma, and cardiovascular diseases. Significant progress on any and all of these problems will be data intensive, with statistics a key element at the core. The goal of this workshop is to stage the statistical challenges and progress towards solutions in a handful of emerging and mission-critical areas of the health sciences with global impact. Specifically, focus will be on the following three areas: digital health, machine learning in causal inference, and networks for public health. Ultimately, the idea is to bring together a gathering of representatives from statistics and related domain areas, in an agile and interactive format, and use a web-based dissemination platform to bring broad visibility to these topics.
Agenda
8:00 a.m. – 8:30 a.m.
DOORS OPEN, BREAKFAST AVAILABLE
8:30 a.m. – 8:50 a.m.
OPENING REMARKS
President, Boston University
Dean and Robert A. Knox Professor, Boston University School of Public Health
Professor and Chair, Department of Biostatistics, Boston University School of Public Health
8:50 a.m. – 9:50 a.m.
PLENARY SPEAKERS
Susan Murphy
Professor of Statistics, Radcliffe Alumnae Professor at the Radcliffe Institute, Harvard University and, Professor of Computer Science at the Harvard John A. Paulson School of Engineering and Applied Sciences
Read More
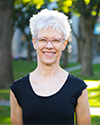
Susan Murphy is a Professor of Statistics, Computer Science and Radcliffe Alumnae Professor, Harvard University. Dr. Murphy’s lab develops data analysis methods and experimental designs to improve real time sequential decision-making in mobile health. In particular her lab develops algorithms, deployed on wearable devices, to deliver and continually optimize individually tailored treatments. She developed the micro-randomized trial for use in constructing mobile health interventions; this trial design is in use across a broad range of health related areas. In these trials each participant can be randomized or re-randomized 100’s of times. Examples of micro-randomized trials that are completed or are in the field can be found at
https://methodology.psu.edu/ra/adap-inter/mrt-projects#proj.
Dr. Murphy is a member of the National Academy of Sciences and of the National Academy of Medicine, both of the US National Academies. In 2013 she was awarded a MacArthur Fellowship for her work on experimental designs to inform sequential decision making.
Joseph Lehar
VP Data Science Analytics & Insights, The Janssen Pharmaceutical Companies of Johnson & Johnson, Boston University, Adjunct Assistant Professor, Bioengineering & Bioinformatics, Boston University
Read More

Joseph Lehar has been working in drug discovery for over 15 years after moving from his first career in astrophysics. Prior to Janssen and Merck, he built computational biology teams at Google/Verily, Novartis, and CombinatoRx. His research experience touches on systems biology, high-throughput screening, clinical investigations, and digital phenotypes in oncology and other disease areas. Please see Google Scholar for a current list of publications.
9:50 a.m. – 10:00 a.m.
BREAK
10:00 a.m. – 11:10 a.m.
PART ONE: DIGITAL HEALTH: INTEGRATING WEARABLES, SOCIAL MEDIA, AND MORE
Keynote
Vadim Zipunnikov
Assistant Professor, Department of Biostatistics, Johns Hopkins Bloomberg School of Public Health
Read More

Dr. Vadim Zipunnikov is an Associate Professor in the Department of Biostatistics at the Johns Hopkins School of Public Health. Dr. Zipunnikov is a faculty member of Statistical Methodology and Applications in Technology (SMART) group, a co-leader of the Wearable and Implantable Technology (WIT) group, and the biostatistics co-director of the Motor Activity Research Consortium for Health (mMARCH). His group works on methods development for multi-modal data generated by wearables (such as fitness and sleep trackers, heart rate monitors, and electronic diaries) and interfacing these data with larger databases of more traditionally collected information (by large national epidemiological studies, health surveys, and Electronic Medical Records). He also focuses on the understanding the complex dynamic interactions of multiple measures of sleep, physical activity, and circadian rhythmicity coupled with the context from electronic diaries to characterize multi-modal behavioral phenotypes via deeper understanding of the dynamic interplay of multiple brain-body systems and engineering of interpretable and predictive biomarkers.
Panelists
Pei Wang
Associate Professor, Genetics and Genomics Sciences, Icahn School of Medicine at Mount Sinai
Read More

Dr. Wang is a Professor of Genetics and Genomic Sciences in Icahn school of Medicine at Mount Sinai, New York. She obtained her B.S. in Mathematics from Peking University, China, in 2000. She then received her Ph.D. in Statistics from Stanford University in 2004. Between 2004-2013, Dr. Wang served as a faculty at Fred Hutchinson Cancer Research Center and University of Washington, Seattle, WA. In Oct 2013, she joined Icahn Medical School at Mount Sinai, New York. Dr Wang’s research focuses on data analysis of high dimensional omics and medical data. Together with her collaborators, Dr. Wang has developed a large number of novel machine learning statistical methods for analyzing CNV arrays, SNP arrays, next generation sequencing data, mass spectrometry based proteomics data, and many other omics data. Dr. Wang also has made substantial contribution to high dimensional network inference. She and her group has developed a series of statistical/computational methods for constructing high dimension gene regulatory networks, as well as utilizing network tools to integrate different biology data sets. Right now, Dr. Wang is leading a proteogenomic data analysis center for CPTAC (Clinical Proteomic Tumor Analysis Consortium) of NCI. Dr. Wang is also directing the data scientist group for the Center of Digital Health, Icahn Medical School at Mount Sinai.
Greg Hather
Scientific Fellow, Takeda Pharmaceuticals
Read More

Greg Hather obtained his Ph.D. in Statistics at the University of California, Berkeley in 2008. Since then, he has worked at Seattle Children’s Research Institute, Pfizer, and Takeda Pharmaceuticals. Currently, he is a Scientific Fellow at Takeda, where he has performed analysis for a variety of functions, including discovery, assay development, manufacturing, and outcomes research. Since 2017, Greg has supported Takeda’s digital trials. He has contributed to the design and analysis of eight different clinical trials that included wearables or apps. Greg’s research interests include novel methods to assess the sensitivity of digital measurements to detect changes in health status. In addition, he seeks to adapt clinical trial designs and analysis methods to make use of frequent digital measurements.
Professor and Data Science Faculty Fellow, Department of Computer Science, Boston University
Assistant Professor and Data Science Faculty Fellow, Boston University School of Public Health
11:10 a.m. – 12:20 p.m.
PART TWO: MACHINE LEARNING IN CAUSAL INFERENCE
Beth Ann Griffin
Senior Statistician, Co-Director RAND Center for Causal Inference, RAND Corporation
Read More

Beth Ann Griffin is a senior statistician at the RAND Corporation. Her research has largely focused on causal effects estimation when using observational data. Her substantive research has primarily fallen into three areas: (1) substance abuse treatment for adolescents, (2) the impact of non-genetic factors on Huntington’s disease, and (3) the effects of gun and opioid state policies on outcomes. She co-directed the RAND Center for Causal Inference between 2013-2018. Currently, Griffin is a co-investigator on Gun Policy in America, a RAND initiative to understand the effects of gun policies, and is co-directing RAND’s Opioid Policy Tools and Information Center (OPTIC) to foster innovative research, tools and methods for tackling the opioid epidemic.
She is the principal investigator on two grants sponsored by the National Institute of Drug Abuse (NIDA), one focused on developing new tools and methods to understand causal mediation and moderation and assess the sensitivity of effect estimates to omitted variables and another which aims to develop well-operationalized, empirically-supported sequences of decision rules—known as “Adaptive Interventions” (AIs)—to provide guidance about substance-use services decisions for adolescent clients. Griffin’s research has appeared in leading journals such as Drug and Alcohol Dependence, Statistics in Medicine, Journal of the American Statistical Association, Annals of Applied Statistics, Journal of Causal Inference, and American Journal of Public Health. Griffin also serves on the editorial board of the Annals of Applied Statistics. She received her PhD in biostatistics from Harvard University.
Susan Gruber
Principal, Putnam Data Sciences
Read More

Susan Gruber is a biostatistician and a computer scientist with a PhD in Biostatistics, a Masters in Public Health, and a Masters in Computer Science. In 2017 She founded Putnam Data Sciences, a statistical consulting firm. Her work focuses on the development and application of data adaptive methodologies for improving the quality of evidence generated by observational health care studies. Dr. Gruber is the former Director of the Biostatistics Center and Assistant Professor in the Department of Population Medicine, Harvard Medical School and Harvard Pilgrim Healthcare Institute. Prior to that she served as Senior Director of IMEDS Methods Research at the Reagan-Udall Foundation for the FDA.
Stefan Wager
Assistant Professor of Operations, Information, and Technology, Stanford Graduate School of Business
Read More

Stefan Wager is an Assistant Professor of Operations, Information and Technology at Stanford University’s Graduate School of Business, and an Assistant Professor of Statistics (by courtesy). He received his Ph.D. in Statistics from Stanford University in 2016, and was a postdoctoral researcher at Columbia University during the academic year 2016-2017. Professor Wager’s research lies at the intersection of causal inference, optimization, and statistical learning. He is particularly interested in developing new solutions to classical problems in statistics, economics and decision making that leverage recent developments in machine learning.
Laura Balzer
Assistant Professor of Biostatistics, Department of Biostatistics & Epidemiology, School of Public Health & Health Sciences, University of Massachusetts – Amherst
Read More

Dr. Laura Balzer is an Assistant Professor of Biostatistics at the University of Massachusetts-Amherst. She earned her PhD from the University of California-Berkeley and completed her post-doctoral studies at the Harvard School of Public Health. Her areas of expertise include Causal Inference and Machine Learning. Her research focuses on challenges arising from studies with complex measurement, missingness, and dependence. Dr. Balzer is the Primary Statistician for three cluster randomized trials in East Africa: the SEARCH study to prevent HIV and improve community health, the SATURN study to improve care outcomes among HIV+ youth, and the SPIRIT study to prevent tuberculosis. Her work is supported by the National Institutes of Health (NIH), and has been recognized with the ASA’s Causality in Statistics Education Award and the Gertrude M. Cox Scholarship.
Assistant Professor, Boston University School of Public Health
12:20 p.m. – 12:50 p.m.
LUNCH
12:50 p.m. – 2:00 p.m.
PART THREE: NETWORKS FOR PUBLIC HEALTH
Key Lecture
David Dunson
Arts and Sciences Professor of Statistical Science, Duke University
Read More

David Dunson is Arts and Sciences Distinguished Professor of Statistical Science and Mathematics at Duke University. He is known for his wide-ranging contributions to statistical methodology, with a particular focus on novel modeling frameworks and Bayesian approaches motivated by complex and high-dimensional data collected in the sciences. These includes latent factor, dimensionality reduction, nonparametric and machine learning methodology. Primary application areas of his work include neurosciences and brain network modeling, environmental health, ecology, and human fertility among others. He is a fellow of the ASA, IMS and ISBA and has won numerous awards, including most notably the 2010 COPSS President’s Award. His work is very widely cited, and he has an H-index of 72 on Google scholar.
Panelists
Tian Zheng
Professor, Department of Statistics, Columbia University
Read More

Tian Zheng is Professor of Statistics at Columbia University and Associate Director for Education of Columbia’s Data Science Institute. She obtained her PhD from Columbia in 2002. She develops novel methods for exploring and understanding patterns in complex data from different application domains such as biology, psychology, climatology, and etc. Her current projects are in the fields of statistical machine learning, spatiotemporal modeling and social network analysis. Professor Zheng’s research has been recognized by the 2008 Outstanding Statistical Application Award from the American Statistical Association (ASA), the Mitchell Prize from ISBA and a Google research award. She became a Fellow of American Statistical Association in 2014. Professor Zheng is the receipt of 2017 Columbia’s Presidential Award for Outstanding Teaching. In 2019, she is the section chair for ASA’s section on Statistical Learning and Data Science.
Assistant Professor, Department of Sociology, Boston University
Ali Shojaie
Associate Professor, Department of Biostatistics, University of Washington
Read More

Ali Shojaie is an Associate Professor of Biostatistics and Adjunct Associate Professor of Statistics at the University of Washington. Originally trained in Industrial and Systems Engineering, he obtained his PhD in Statistics from the University of Michigan, while completing Masters degrees in Applied Mathematics and Human Genetics. Dr. Shojaie’s research lies in the intersection of statistical machine learning, statistical network analysis and applications in biology and social sciences. He teaches multiple regular and short courses on statistical machine learning and network analysis, and is the founding director of the Summer Institute for Statistics in Big Data (SISBID).
Assistant Professor, Boston University School of Public Health
2:00 p.m. – 2:20 p.m.
CLOSING REMARKS
Bhramar Mukherjee
John D. Kalbfleisch Collegiate Professor of Biostatistics and Chair of Biostatistics, The University of Michigan School of Public Health
Read More

Bhramar Mukherjee is John D. Kalbfleisch Collegiate Professor and Chair, Department of Biostatistics; Professor, Department of Epidemiology, Professor, Global Public Health, University of Michigan (UM) School of Public Health; Research Professor and Core Faculty Member, Michigan Institute of Data Science (MIDAS), University of Michigan. She also serves as the Associate Director of Cancer Control and Population Sciences, The University of Michigan Rogel Cancer Center. She is the cohort development core co-director in the University of Michigan’s institution-wide Precision Health Initiative. Her research interests include statistical methods for analysis of electronic health records, studies of gene-environment interaction, Bayesian methods, shrinkage estimation, analysis of multiple pollutants. Collaborative areas are mainly in cancer, cardiovascular diseases, reproductive health, exposure science and environmental epidemiology. She has co-authored more than 200 publications in statistics, biostatistics, medicine and public health and is serving as PI on NSF and NIH funded methodology grants. She is the founding director of the University of Michigan’s summer institute on Big Data. Bhramar is a fellow of the American Statistical Association and the American Association for the Advancement of Science. She is the recipient of many awards for her scholarship, service and teaching at the University of Michigan and beyond.
Professor and Data Science Faculty Fellow, Department of Mathematics and Statistics, Boston University