Advancing Assistive Technologies with AI
BY ZOE TSENG
For someone who is visually impaired, navigating an unfamiliar street can be challenging. Even going straight can be tough in an open space. Encountering obstacles, stairs, and intersections can potentially result in an unsafe situation. While aids, such as white canes or guide dogs are helpful, they can’t exactly tell someone who is visually impaired what is in front of them or where to go.
Professor Eshed Ohn-Bar works on developing AI technologies that can seamlessly collaborate and help humans. Ohn-Bar is a Hariri Institute Junior Faculty Fellow and Thrust Leader of the AI for Assistive Applications and Natural Interfaces, part of the Focused Research Program Teaching Machines Human-Like Intelligence. He is also a CISE faculty affiliate and Professor of Electrical and Computer Engineering at the College of Engineering.
Ohn-Bar’s most recent paper, “ASSISTER: Assistive Navigation via Conditional Instruction Generation,” introduces a language generation AI for providing intuitive, human-like assistance to individuals with visual impairments. The goal is for ASSISTER to be able to determine what and when to tell a person who is visually impaired, such as what obstacles they are facing, while verbally directing the person to their destination by planning out a path.

“There’s a grand engineering challenge of assisting individuals with visual impairments to navigate and get to their destination safely and seamlessly,” Ohn-Bar said. “Individuals with disabilities often say that transportation and getting to places can affect their quality of life because they may want to go to restaurants or the gym. But, they may end up staying home because they feel it’s too difficult to get to these places independently.”
Ohn-Bar and his team wanted the ASSISTER AI to act less like automated guidance, and instead be more natural and geared towards the person’s own interpretation of directions and abilities.
“The goal of ASSISTER is to mimic natural human interaction as opposed to providing feedback similar to an autocorrect system that gives you some recommendation you don’t want to use,” Ohn-Bar said.
To mimic more natural interactions, Ohn-Bar and his team hired orientation and mobility guides. These guides work with individuals who are visually impaired to teach them how to navigate to a destination. While they were navigating, the travelers wore cameras, allowing the researchers to observe how the guides gave directions and their responses. They then took the video and audio data from those experiences and used it to train ASSISTER. Using a speaker-follower model, ASSISTER was programmed to provide conversational instructions through a wearable assistive system.
Ohn-Bar and his team tested ASSISTER in a simulation first, over diverse scenarios and environments, and then with humans following instructions in the real world. The simulation mimics what it’s like to navigate without being able to see anything. The user can use keyboard keys to move around, but all they see on the screen is a colored fan indicating the position of their cane. With just the visual of the fan on the screen, the user must complete the task of finding their rideshare vehicle.
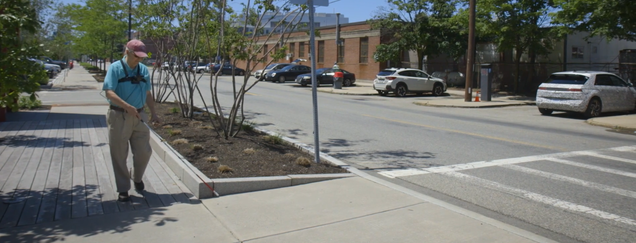
Once they fine-tuned the algorithm, they then transferred the AI to the real-world. Ohn-Bar and his team collaborated with the Carroll Center for the Blind in Boston, with users given instructions to reach an autonomous vehicle 200ft away across busy intersections, stairs, curbs, and pedestrians. The step-by-step assistive system was able to guide users all the way to the door handle of the ride.
Ohn-Bar said that while he always worked on human-machine interaction for assistive technologies, working with a blind computer scientist at Carnegie Mellon University showed him first-hand how technology can alleviate difficulties of individuals who are blind. He also mentioned how his grandmother lost much of her sight a few years ago.
In general, AI and computer vision today has a difficult time understanding humans with disabilities. In a paper titled X-World: Accessibility, Vision, and Autonomy Meet, Ohn-Bar and his team discovered that algorithms detected a wheelchair with 30% accuracy, and white canes with less than 1% accuracy, showing there is a lack of research in teaching machines to recognize and interact with individuals with disabilities.
Ohn-Bar and his team recently received an NSF grant to continue the research with ASSISTER. They are currently testing a smartphone app version of ASSISTER and Ohn-Bar said he wants ASSISTER to turn into a complete trip support system that anyone can use. Essentially, the app would guide anyone from one destination to another, and then back home. He hopes his work will make it easier for people with disabilities to navigate and achieve everyday tasks with ease.