Focused Research Program Faculty Member Awarded Funding from The Camille and Henry Dreyfus Foundation
BY GINA MANTICA
When you take medicine, the drug moves its way through your body until it attaches to parts of proteins that are capable of achieving the drug’s desired effect. But figuring out which part of a protein a drug should target can be challenging: one small mutation in a protein can cause a huge change in the protein’s structure and affect how the drug binds. Artificial intelligence experts are teaming up with chemists to better understand the relationship between the structure and function of proteins’ binding sites.
Qiang Cui, a Professor of Chemistry and Research Fellow at the Hariri Institute, received a 2021 Machine Learning in the Chemical Sciences & Engineering Award from The Camille and Henry Dreyfus Foundation to develop a machine learning approach for analyzing protein allostery, or how the activity of a protein changes depending on how other molecules binding to it. The project, entitled, “Understanding Protein Allostery using Machine Learning and Deep Mutation Data”, stems from conversations Cui had with other experts in the Machine Learning for Chemistry & Materials Science Focused Research Program (FRP) about how to improve the robustness of a model developed with a collaborator at University of Wisconsin-Madison.
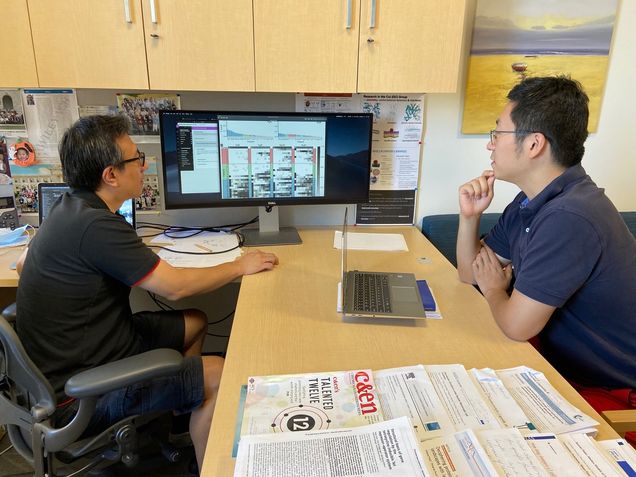
The model that Cui’s team developed uses experimental data about the structure of proteins to predict whether a mutation would change the protein’s allosteric functionality. But the model can only analyze single mutations. Cui wants to improve the model by incorporating sequence information of related proteins, so that researchers can better predict the function of a protein with multiple mutations.
Pharmaceutical researchers take advantage of protein allostery to design drugs that bind to different parts of a protein, increasing a drug’s specificity and effectiveness. Machine learning enables researchers to extract meaningful information from large experimental data sets on how complex mutations can affect a protein’s structure and function, aiding in the discovery of new drugs.
The Hariri Institute’s FRPs catalyze transformative research projects that enable new methodologies, advancements, and technologies. “The tools we [Cui’s team] use in this project are relatively established machine learning techniques, but we are not experts to improve the effectiveness and robustness of these tools,” said Cui, “The FRP was very useful in terms of introducing us to local machine learning experts.”
Interested in learning more about the research happening at the Hariri Institute? Sign up for our newsletter here.