Event Recap: Machine Learning for Model-Rich Problems
BY: NATALIE GOLD
Machine learning has quickly emerged as an important innovation in artificial intelligence (AI) and computer science and over time, has been applied across other fields and disciplines. During the month of April, the Hariri Institute brought together four speakers from across different fields who have used machine learning in their work as part of the Distinguished Speaker Series: “Machine Learning for Model-Rich Problems”. The speakers came from backgrounds ranging from biophysics to economics, and showed how machine learning tools can be used to enhance research in their fields.

On April 16, Cecilia Clementi, Professor of Physics & Researcher at Freie Universität Berlin, presented her talk, “Designing Molecular Models with Machine Learning and Experimental Data.” Clementi spoke about coarse grained modeling, a molecular modeling tool. Molecular systems can be difficult to study because of their interconnected nature, and small, hard to understand details. Coarse grained models simulate the behavior of these complicated systems in a simpler way, and allow for longer simulation times to be studied with slightly less specific molecular detail. There are different methods of creating coarse grained models. A scientist chooses the method depending on the data they have and how much detail they want included in the model. Celementi spoke specifically about bottom up modeling, the classical atomistic method that uses deep learning to develop a model that reproduces the same thermodynamic properties, or the same free energy, as in simulations. By utilizing coarse grained modeling, researchers can understand small biological systems that cannot be studied the same way that larger proteins are.

On April 22, Miguel A. Bessa, Associate Professor in Materials Science and Engineering at Delft University of Technology, gave his talk “Machine Learning Advances in Mechanics of Materials.” Bessa addressed how researchers can accelerate the analysis and design of materials and structures by using a data driven framework. This framework takes data from computational analysis and applies it to machine learning to create models that predict how materials will behave depending on characteristics, such as the composite of materials. This allows researchers to better analyze materials. One challenge to this framework is using material that has imperfection sensitivity, a sensitivity to stress that would cause materials to buckle, making a material unstable and able to rapidly change state. For these materials, researchers can use Bayesian machine learning, a method that predicts the average behavior of different designs and situations. Despite challenges in using a data driven framework, there are ways to push forward so that all areas of mechanics benefit from the improved analysis provided by machine learning.

On April 26, Jacob Bortnik, Professor in Atmospheric and Oceanic Sciences at University of California, Los Angeles, presented his talk “(Machine) Learning to Live Near a Temperamental Star.” As it has become cheaper to get to space, more organizations are sending spacecraft into orbit. Each of these thousands of satellites provide large amounts of data. However, for this data to be useful, scientists must keep up with their analysis. This is where machine learning is helpful. Machine learning allows scientists to take large amounts of data from the satellites, and create models to understand the dynamics of the space environment they are in. Data can also be used to create machine learning models that predict space weather, which allows scientists on earth to make sense of data coming from the atmosphere hundreds of miles away and better understand an environment they would otherwise have no access to.
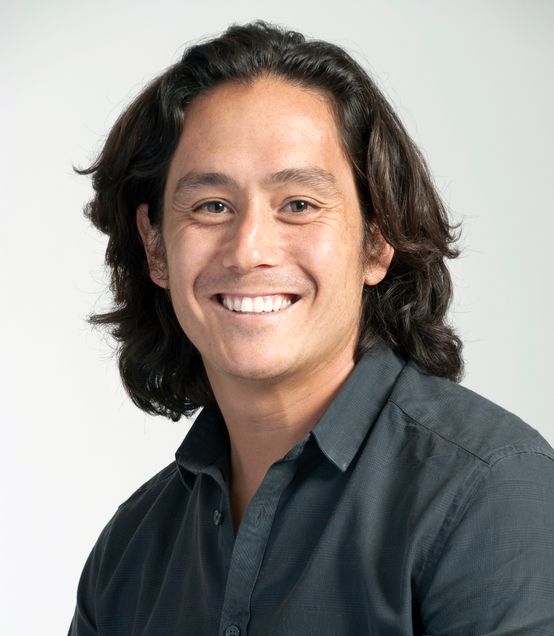
On April 30, Joshua Blumenstock, Associate Professor at University of California, Berkeley’s School of Information, gave his talk “Machine Learning Approaches to Targeting Emergency Humanitarian Assistance.” In the past few years, many countries have experienced a “hunger pandemic”. In response to hunger and poverty issues, countries implement targeted social assistance programs that get money to those who most need it. Determining who has the highest need of assistance can be challenging in low and middle income countries that don’t have updated data on individual’s income. Machine learning can be used to overcome this lack of data. Satellite data and imagery can be analyzed by machine learning algorithms to recognize features that would be most indicative of poverty, such as unpaved roads or metal roofs. Individuals can also be targeted with cell phone data, based on differences in how ultra-poor and non ultra-poor individuals use their phones. Machine learning models can be trained on data linking socioeconomic status and cell phone use to determine which individuals are the poorest in a region. Machine learning models may be able to help governments in middle and low income countries target social assistance more effectively.
At the end of the series, the four speakers participated in a panel to discuss where data-driven modeling interfaces with model-rich fields. Although all four speakers come from different backgrounds, they found similarities in how they use machine learning tools in their work to better make sense of large amounts of data. In this way, machine learning facilitates integration and convergence of scientific disciplines. The four speakers also discussed how machine learning can be used to find solutions to problems in ways that are not possible with other theories and tools. As understanding of machine learning continues to develop, researchers from across disciplines will find more ways to better understand data and discover solutions to problems facing the world.