Some Empirical Observations Linking Representation and Generalization

Samy Bengio, Google
When:
Monday, February 4, 2019
Networking reception, 10:30-11:00am; Seminar 11:00-12:00pm
Where:
Photonics Building: 8 St. Mary’s Street, 9th Floor, Colloquium Room
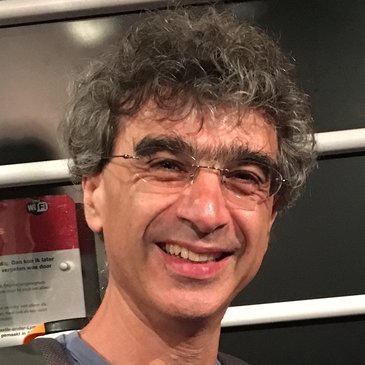
Abstract: Deep learning has shown incredible successes in the past few years, but there is still a lot of work remaining in order to understand some of these successes. Why do such over-parameterized models still generalize so well? In this presentation, I will cover a few of my recent work empirically showing interesting relations between learned internal representations and generalization. Note that this covers joint work with many of my colleagues at Google Brain.
Bio: Samy Bengio (PhD in computer science, University of Montreal, 1993) is a research scientist at Google since 2007. He currently leads a group of research scientists in the Google Brain team, conducting research in many areas of machine learning such as deep architectures, representation learning, sequence processing, speech recognition, image understanding, large-scale problems, adversarial settings, etc. He is the general chair for Neural Information Processing Systems (NeurIPS) 2018, the main conference venue for machine learning, was the program chair for NIPS in 2017, is action editor of the Journal of Machine Learning Research and on the editorial board of the Machine Learning Journal, was program chair of the International Conference on Learning Representations (ICLR 2015, 2016), general chair of BayLearn (2012-2015) and the Workshops on Machine Learning for Multimodal Interactions (MLMI’2004-2006), as well as the IEEE Workshop on Neural Networks for Signal Processing (NNSP’2002), and on the program committee of several international conferences such as NIPS, ICML, ICLR, ECML and IJCAI. More information can be found on his website.