Using Big Data, Machine Learning to Reduce Chronic Disease Spending
Researchers are using machine learning and big data to reduce healthcare spending on chronic conditions, including diabetes and heart disease.
By Jessica Kent, originally published on HealthITAnalytics.com 8/8/18
Diabetes and heart disease are two of the most costly and prevalent chronic conditions impacting patients in the US, leading the healthcare industry to spend billions every year to treat and manage these disorders.
As care delivery continues to evolve from reactive disease treatment to proactive, preventive care, more organizations are looking to advanced technologies like artificial intelligence and machine learning to assist with drawing actionable conclusions from their big data resources.
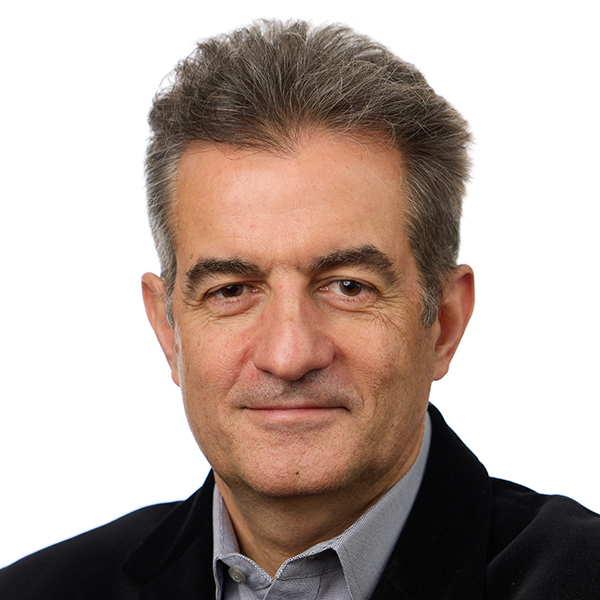
“There is a lot of information about every one of us, in EHRs, in smart phones, in smart watches, and in other tracking devices,” Iaonnis Paschalidis, Professor of Engineering and Director of the Center for Information and Systems Engineering at Boston University, told HealthITAnalytics.com.
“We can now analyze what happens to patients in real time and characterize the status and health condition of each individual.”
Machine learning and AI approaches will prove invaluable for predicting events such as hospitalizations and readmissions, both of which account for a great deal of healthcare spending, Paschalidis noted.
“An AHRQ study estimates that on an annual basis, the US spends about $30 billion on hospitalizations that could have been prevented,” he said.
“And the majority of those costs, about 50 percent, are due to chronic diseases. About 30 percent of that $30 billion is due to heart disease, and another 20 percent is due to diabetes. These are hospitalizations that could have been prevented with better care, and that’s a significant amount that could have been spent in other areas of need.”
To help overcome these challenges and reduce unnecessary spending, Paschalidis is collaborating with Boston Medical Center (BMC).
The two organizations will use a three-year, $900,000 grant from the National Science Foundation to develop machine learning algorithms that will identify patients at higher risk of heart disease or diabetes. Researchers will use the algorithms to enable early interventions and personalized treatments for high-risk patients.
“With machine learning methods, we can process very large amounts of data and examine the many factors that are involved in predicting a future outcome,” Paschalidis said.
“We’re aiming to deliver personalized predictions and personalized recommendations to individuals, with the goal of improving outcomes and reducing costs.”
This project will build on earlier work conducted by Paschalidis and his team, in which they used patients’ electronic health records (EHRs) and machine learning to predict hospitalizations due to diabetes and heart disease.
Using this method, the group found that they could predict hospitalizations about a year in advance, with an accuracy rate of up to 82 percent.
Now, Paschalidis and his team will develop even more comprehensive predictive capabilities using EHRs and real-time health data, including information from wearables, implantable devices, and home-based networked diagnostic devices.
“When we use machine learning methods that can look at 200 variables instead of five or six variables, there’s a very significant jump in terms of accuracy,” he said.
Researchers will also use machine learning to optimize chronic disease management and treatment protocols for these conditions.
Current treatment protocols typically utilize a one-size-fits-all approach, assessing the stage of disease rather than the individual patient.
With machine learning algorithms, however, providers can design personalized interventions for each patient, from increased monitoring to modified treatment plans. These tailored efforts can help them intervene before a patient’s condition reaches a critical phase, resulting in lower care costs and improved health outcomes.
The project will pilot the algorithms by integrating them into EHRs at 14 community health centers, including BMC.
“BMC is a large safety net hospital, whose goal is to care for the lower-income, minority, and underrepresented communities in the Boston area,” Paschalidis said.
“A major aspect of the project is to collaborate with BMC, and to ensure the algorithms we develop are useful tools for physicians when taking care of their patients.”
Introducing these algorithms to BMC and other health centers can potentially help these organizations make the transition to preventive, proactive care.
Going forward, Paschalidis believes that machine learning will most benefit chronic disease care and population health management.
“If you look at the current state of healthcare in the US, we are extremely good at treating conditions when they appear, and when these conditions become serious enough to warrant attention by the healthcare system. However, I don’t think we are so good in terms of preventing these conditions,” he said.
“Using machine learning to come up with personalized predictions and recommendations will significantly strengthen prevention efforts. Better prevention can not only improve outcomes for individuals, but also substantially reduce healthcare spending.”
In order to achieve this goal, however, machine learning tools must be trustworthy, accurate, and rooted in reliable data, he cautioned.
“One potential risk of using machine learning is that the data could be inaccurate. If we are training algorithms that are making predictions on inaccurate data, there is a risk that we could reach the wrong conclusions,” Paschalidis said.
“We and others are doing quite a bit of work in developing what we call robust learning methods. These are more sophisticated machine learning methods that can accommodate outliers and errors in the data and correct them.”
With this new project and collaboration with BMC, Paschalidis and his team will target two of the costliest chronic conditions and develop personalized treatment plans to boost outcomes and reduce spending.
“We have looked at heart disease and diabetes, these being perhaps the two most important chronic diseases that affect the most people,” Paschalidis said.
“The project will give us the resources to support and partner with BMC to develop algorithms that will give the healthcare system enough time to act and potentially prevent adverse patient outcomes.”