Can We Harness AI?
Making AI work for us all.
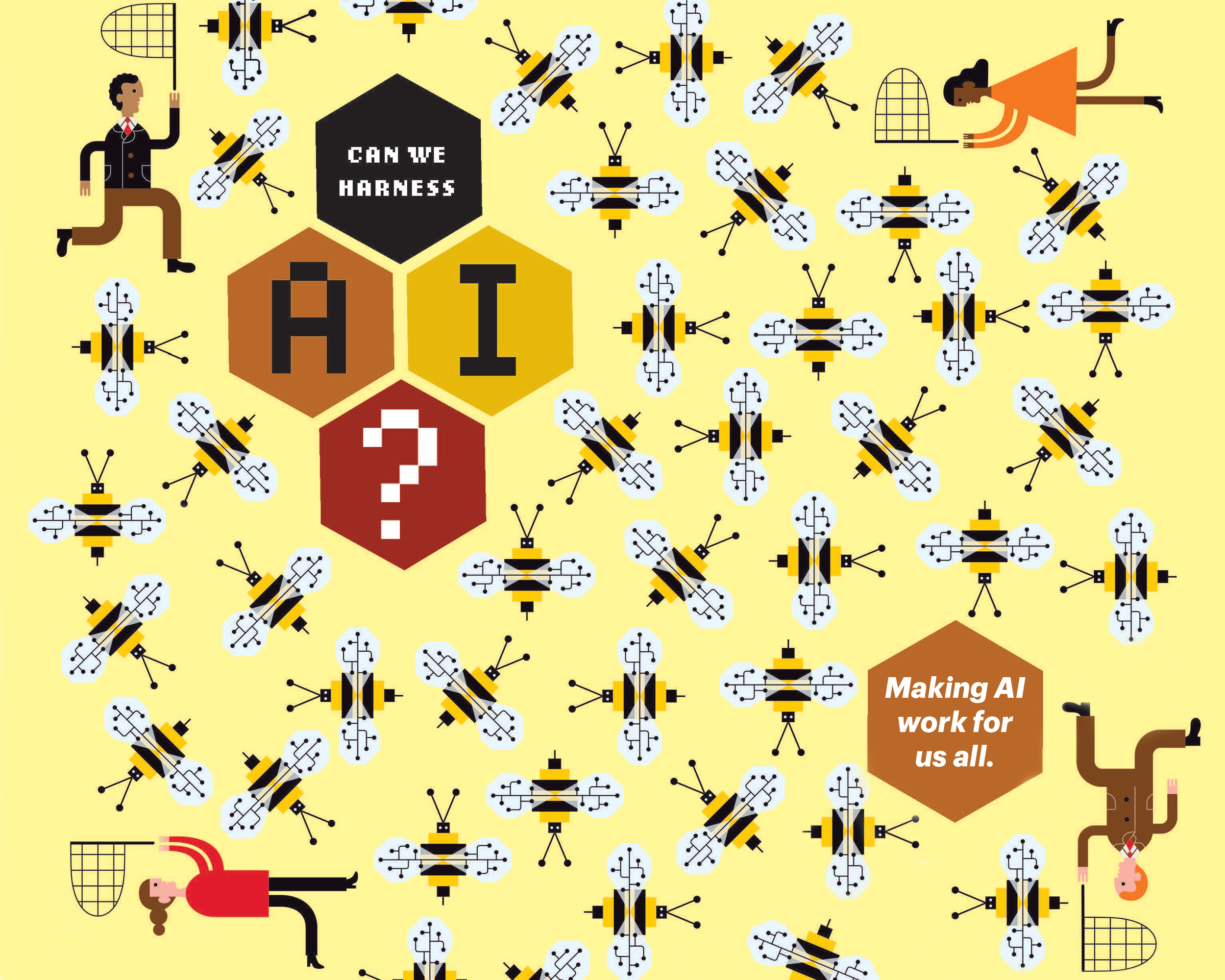
Early in his career as an academic, Boston University School of Law Professor Woodrow Hartzog codesigned an experiment in which computer science students created algorithms to enforce a 55-mile-per-hour speed limit.
The speed restriction was chosen for its simplicity: a person either drove above 55 miles per hour or not. And yet, the students’ efforts yielded wildly disparate results. One group, assigned to implement the “letter of the law,” issued 498 tickets in a 66-minute drive where the driver rarely exceeded the speed limit (and never by more than 10 miles per hour). Another group, tasked with implementing the “intent of the law,” issued only one ticket using data from the same drive.
Perhaps even more significant was how the students felt about their work. When asked whether they would want their driving to be monitored by the algorithmic systems they designed, the overwhelming answer was no.
“Our conclusion was, let’s not rush into automating enforcement of laws just yet,” Hartzog remembers.
That was 10 years ago. Today, all kinds of entities in the private and public sectors are rushing into automation and artificial intelligence (AI) with seemingly little regard for the consequences, including in law enforcement contexts. The pace is so dizzying that even the people behind some of the most prominent and dominant AI-focused companies—like Microsoft President Brad Smith and Sam Altman, chief executive of OpenAI, which created ChatGPT—have called on the government to do more to regulate the ever-evolving technologies.
But what would better regulation of AI look like? Answering that question will require new ways of thinking about the law and technology. Hartzog and other BU Law faculty are at the forefront of that thinking, part of a new generation of interdisciplinary scholars intent on finding ways to secure AI’s benefits without the whole of society succumbing to its harms.
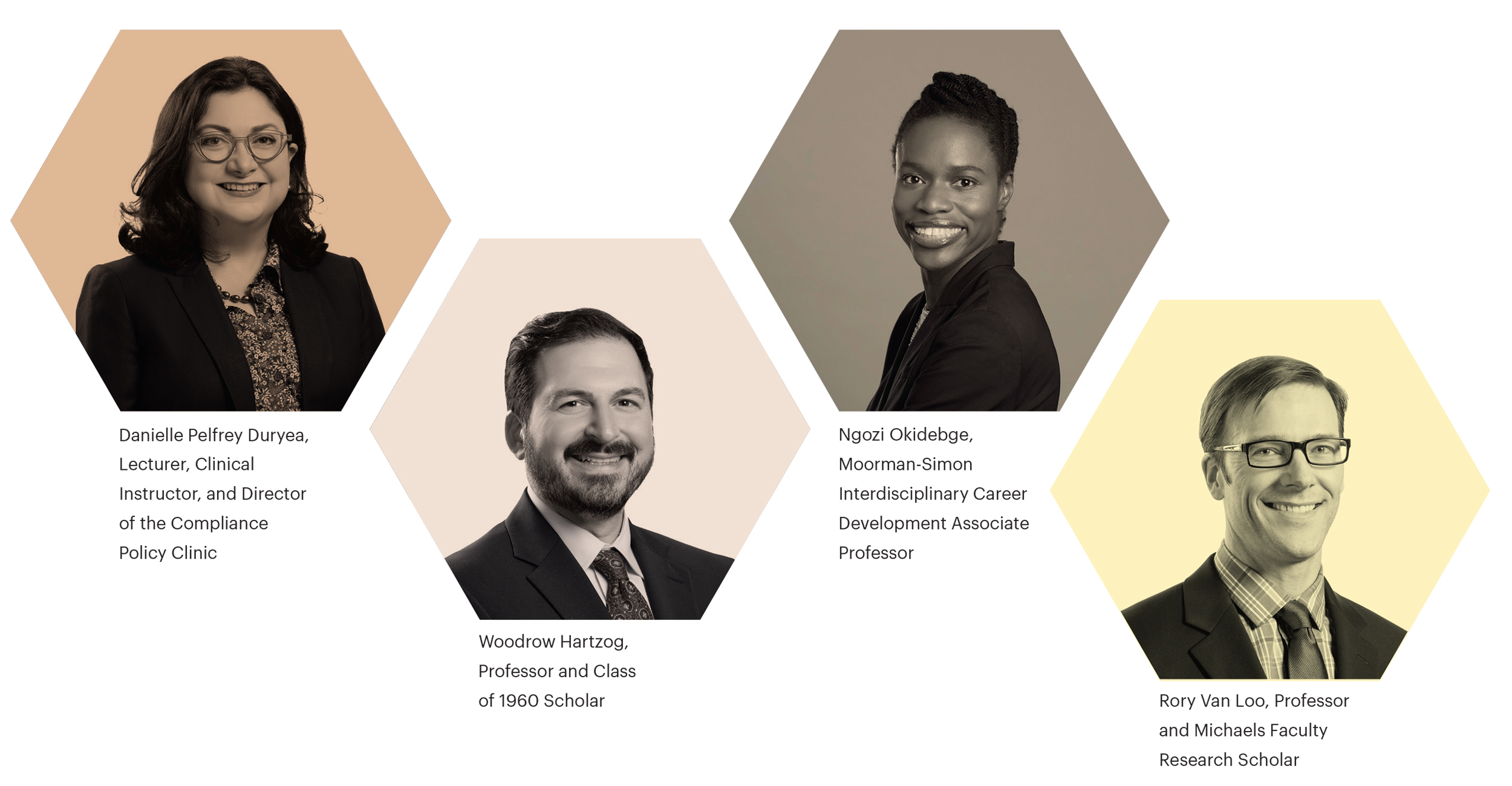
“Issues of law and technology simply cannot be solved by either lawyers or technologists or social scientists or economists alone,” says Hartzog. “Only by bringing all of those people together can we really make meaningful progress.”
Expanding Notions of Harm
Associate Professor Ngozi Okidegbe’s work focuses on the area of the law in which people—and especially historically marginalized communities—have the most to lose if technology gets things wrong: the criminal legal system.
Okidegbe’s scholarship moves beyond the now-well-documented reality that algorithms in the criminal context, including pretrial decisions involving bail, surveillance, and detention, are not neutral or objective, as they were once touted to be. Instead, they perpetuate existing biases. A ProPublica report from 2016 found that Black defendants were almost twice as likely as white defendants to be flagged as potential reoffenders, and that white offenders were more often mislabeled as low risk than Black offenders.
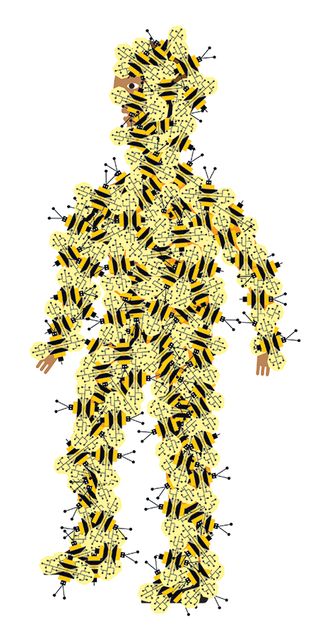
In her work, including “The Democratizing Potential of Algorithms?” and “Discredited Data,” Okidegbe points out that pretrial algorithms are created, adopted, and implemented without input from the communities most impacted by their use. They also rely entirely on data sets from “carceral sources”—such as the police, pretrial service agencies, and the courts—in part because that kind of data is readily available to them in aggregated and anonymized form. Okidegbe argues developers should reduce their reliance on data from the criminal system and incorporate data from non-carceral sources, including community groups affected by the carceral system, such as current and formerly incarcerated people.
The bail system is designed to protect public safety, and one problem with relying on carceral data for algorithms in that context, she points out, is that harms to public safety are defined by the carceral system and its officials: Will someone fail to appear for their hearing? Will they commit a crime while they are on pretrial release?
But as people who have been incarcerated or have family members who have been incarcerated know, there are many, many more types of harms to public safety to consider, including the separation of parents from their children, the loss of a job that supports a family, or the dehumanizing effects of detention. For instance, as Okidegbe points out in “Discredited Data,” bail judges have historically used their discretion to presumptively release a woman who is a primary caregiver to a minor child.
“Algorithms are supposed to help us achieve the public safety function of bail,” she says. “But algorithms as currently constructed fail to account for how incarceration can harm public safety.”
Okidegbe notes that algorithms aren’t “inherently good or bad” and that bias can be the result of design (data sources, what weight is given to various factors, etc.), implementation (whether a decisionmaker can override the algorithm, for instance), and oversight (such as whether the algorithm is updated to reflect changes in the law). But an early potential entry point for bias is in the formulation of the problem the algorithm is designed to solve, since that “formulation will affect the interplay between the algorithm and existing inequities.” That’s an area where community groups could add a lot of value, she says.
In a forthcoming paper, Okidegbe proposes creating local commissions to study the adoption and use of an algorithmic model in a certain jurisdiction, with representatives chosen from across the relevant geographic area, including from historically marginalized communities.
Okidegbe says she “believes in the potential of algorithms to be part of improving society” and notes that many scholars and activists are working toward that goal, including at Data for Black Lives, the Design Justice Network, and the Ida B. Wells Just Data Lab.
“It might be possible to build and implement algorithms that support the well-being of all, but this potential can only be unlocked by centering the communities most likely to be harmed by algorithmic use,” she says.
Protecting Privacy
Since his experiment with algorithms and speed limits, Hartzog has expanded his focus to other areas of law and technology, including data privacy. In 2018, he wrote Privacy’s Blueprint: The Battle to Control the Design of New Technologies, a book that makes the case for requiring privacy protections in new products.
Hartzog is a fierce critic of the current “notice and consent” framework governing consumers’ relationships with technology companies—many of which incorporate AI features that are trained on or allow the companies to trade on our personal data—which he says is “fundamentally broken.” Under this framework, platforms give us notice of their data use policies, and we check the box saying we agree to those policies, whether or not we have understood or even read them. If we don’t check the box, we can’t use the platform.
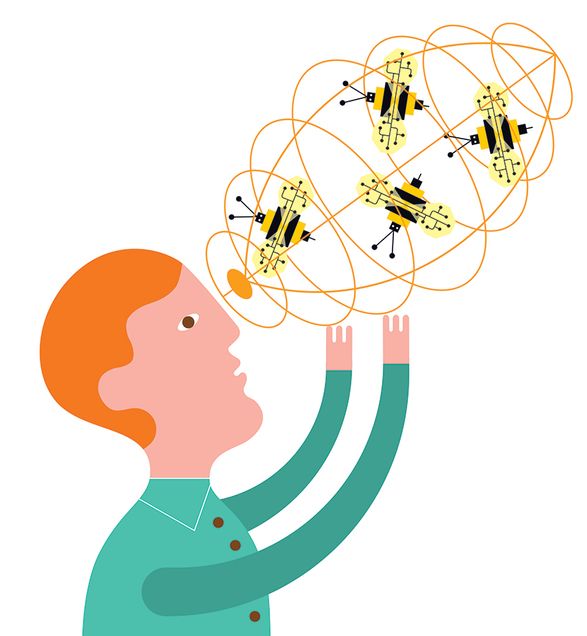
“When you interact with an AI tool or a social media company, you’re extremely vulnerable,” Hartzog says. “You’re at a massive information disparity.”
In “Legislating Data Loyalty,” Hartzog and a coauthor continue to argue that technology companies should instead be governed by a duty of loyalty to their users that would require them to act in our best interests, even when doing so conflicts with their ability to make money.
“We think this is a significantly more productive and sustainable approach to regulating companies dealing with data and information technologies,” he says.
But there’s one AI-powered technology that Hartzog thinks cannot be regulated into safety: facial recognition software.
In a 2018 essay, Hartzog and a coauthor called for an outright ban on the use of facial recognition technology, describing it as an “irresistible tool for oppression.”
“I see no world in which humanity is better off with facial recognition, even with meaningful regulation,” he says.
Several jurisdictions have embraced some sort of ban: In 2019, San Francisco became the first major city to ban government use of facial recognition, and Somerville, Massachusetts, was the first East Coast city to take that step. Portland, Oregon, bans not only government use but also private use in public spaces. In June 2020, Hartzog testified before the Boston City Council in support of an ordinance banning city use of the technology; the ordinance passed later that month.
In Massachusetts, Hartzog served on a statewide body tasked with evaluating use of the technology. In its final report, the Special Commission to Evaluate Government Use of Facial Recognition Technology in the Commonwealth recommended that such software only be used in “limited, tightly regulated circumstances to advance legitimate criminal investigations.”
“I think we were able to reach a compromise…a significant prohibition with limited carveouts for law enforcement and other narrow and justified uses,” he says.
Hartzog argues lawmakers have been complicit in AI- and algorithm-driven privacy violations that harm the public. By failing to confront the technologies head-on with new laws and regulations, he and coauthors argue in “Privacy Nicks: How the Law Normalizes Surveillance,” they have created a surveillance “death spiral.”
“We are all, in some form or another, slow boiling the water we’re sitting in,” he says. “We’ve become accustomed to being watched over the long term in a way that makes it very difficult, if not impossible, to resist the inevitable encroachment of surveillance into our lives.”
Toward Solutions
Countries have taken different approaches to AI regulation. In 2018, the European Union, a perennial early actor in technology regulation, launched the European AI Alliance, which has hosted regular public consultations and engaged thousands of stakeholders; its proposed Artificial Intelligence Act would regulate AI technologies based on their perceived risk. Last spring, China issued draft rules for generative AI products, like ChatGPT, that would prohibit discrimination and false information (but also conform to censors); Italy became the first Western country to ban ChatGPT in March (it later reinstated the service after developer OpenAI announced new privacy controls).
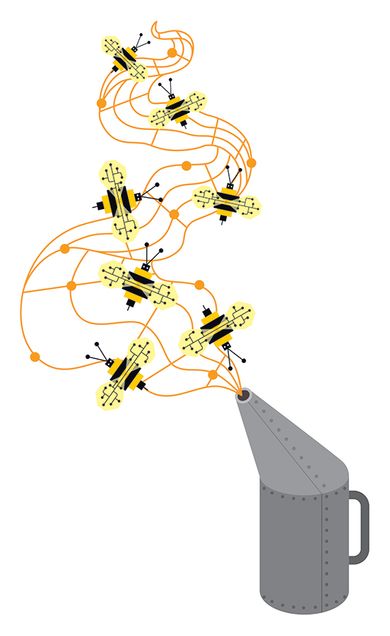
The US has also taken steps toward regulating AI. This fall, the Senate began a series of AI Insight Forums, bringing together lawmakers with technology industry executives and advocacy groups to help Congress create legislation that maximizes the gains and minimizes the risks of AI development and use. Shortly before the first forum, Senators Richard Blumenthal (D-Conn.) and Josh Hawley (R-Mo.) released a framework for AI legislation.
Many of the early steps in the US have come from the executive branch and its agencies. In March, the Copyright Office launched a new initiative to study the copyright law and policy issues raised by artificial intelligence; and in April, a branch of the Department of Commerce invited comments on how to ensure AI accountability. President Joseph Biden signed an executive order directing agencies to prevent algorithmic discrimination, and the administration also announced $140 million in funding to launch several new AI research institutes.
Of course, many existing laws already apply to AI-powered technologies and are being enforced accordingly. In January, the US Department of Justice filed a statement of interest in a case in which two Black women are using the Fair Housing Act to challenge the use of an algorithm-based tenant-screening service that resulted in both women being denied housing. In April, several US entities—the Consumer Financial Protection Bureau, the Department of Justice’s Civil Division, the Equal Employment Opportunity Commission, and the Federal Trade Commission—issued a joint statement on enforcement efforts against discrimination and bias in automated systems.
“There’s always a catch-up kind of aspect to regulation,” says Danielle Pelfrey Duryea, who directs BU Law’s Compliance Policy Clinic. “But it’s not that there is nothing regulating the field. Any consumer protection law, whether at the federal or state level, those are just as applicable to AI as they are to any other product or technology that touches consumers.”
There is also the possibility of taming technology with technology. In “Digital Market Perfection,” Professor Rory Van Loo argues that the law should support so-called digital assistants—think Google Flights—that can search for and eventually even act on lower prices for consumers. AI-powered assistants are necessary, he says, in a world where AI-powered sellers manipulate results so that lower-priced options are harder to find. But some companies have used the Consumer Fraud and Abuse Act and other laws to prohibit third parties from collecting the data that would be necessary to compare prices across companies and brands.
“The sophistication gap is growing between businesses and individual consumers,” Van Loo says. “We need to help consumers have greater sophistication to counterbalance those sales techniques and strategies.”
Van Loo also points out that new regulations may be required to protect against potential unintended consequences of those technologies; for instance, if an AI assistant finds a higher-yield bank account and millions of consumers act on it all at once, that could negatively impact the market.
In other words, in virtually every domain and however artificial intelligence evolves, new regulations and stronger enforcement of existing regulations will probably be necessary and inevitable, the same way environmental and labor laws were enacted as abuses in those areas came to light.
“Every highly regulated industry was once a highly unregulated industry,” Pelfrey Duryea says. “It’s often when pain points appear that governments start moving.”