Summer in the Field: Validating Confidence When Working with Linked Healthcare Data
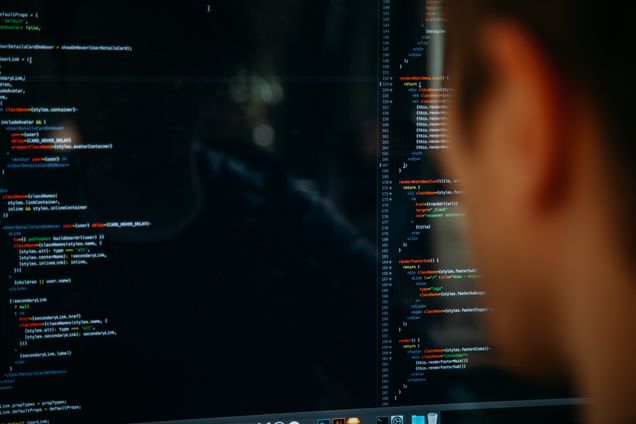
The utilization of large-scale, national-level administrative health data is a very powerful tool to monitor the health of populations. It allows for directly assessing the impact of health policies on a nationwide scale by leveraging the availability of millions of records, eliminating the need for interpolations that are often required when using smaller-scale data. It is especially useful for health policy research, like studying the impact of policy recommendations and messaging campaigns on a broader population level. For instance, in the context of HIV care, administrative data can help researchers evaluate the effectiveness of treatment strategies like the universal test-and-treat for HIV. It also aids in tracking the progress of initiatives like the UNAIDS 95-95-95 targets and efforts to reduce HIV mother-to-child transmission on a national scale.
In situations where patient records lack clear identifiers that distinguish one individual from another (such as patient ID or social security numbers), a common occurrence in large administrative datasets, it can be difficult to track individuals accurately over time. This presents a challenge when attempting to analyze the effectiveness of health policies and interventions over an extended period. For instance, understanding how changes in HIV treatment eligibility impacts engagement in HIV treatment, or understanding the challenges faced by patients in adhering to HIV care, requires a comprehensive, longitudinal patient-level analysis. To tackle this challenge, a common strategy is to use record linkage techniques to create proxy identifiers. These proxy identifiers serve as stand-ins, allowing researchers to infer which records within a dataset likely belong to the same patients. Additionally, this approach helps link patient records spread across different databases.
A major challenge encountered in the record linkage process comes from issues with data quality. Administrative data is primarily collected for purposes other than data analysis, hence there may be less emphasis on ensuring high-quality data. As various individuals contribute to data input, the quality of the data can significantly differ based on the contributor, and errors are common. These inaccuracies often arise from factors such as the use of nicknames, changes in addresses, typographical errors when entering names or birthdates and instances where surnames and first names are mistakenly swapped. As a result, these errors can lead to incorrectly linked records from distinct individuals, wrongly associating them as a single person (referred to as “overmatching”). Conversely, these errors can also result in the failure to link records that pertain to a single person (referred to as “undermatching”).
The inaccuracies that arise during the linking process, known as linkage errors, can significantly affect the precision and reliability of analysis, as well as the level of confidence in research findings. This situation is further complicated by the lack of a standardized method or established guidelines for effectively handling these linkage errors. Consequently, most analyses tend to overlook the impact of these errors when drawing conclusions.
Yet, recognizing and accounting for these errors is crucial. Linkage errors have the potential to sway results, leading to either an underestimation or an overestimation of the actual effects. Furthermore, the extent to which these errors affect findings might not necessarily match the magnitude of the linkage error itself. To illustrate, a 1 percent overmatching rate could exert an influence on the results by more than a few percentage points. It is essential to factor these complexities in when interpreting research outcomes to ensure that conclusions are well-founded.
As part of my Summer in the Field Fellowship, I collaborated with the National Health Laboratory Service (NHLS) in South Africa to develop a user-friendly, generalizable and adaptable validation algorithm. This process is structured in two key steps.
In the first step, the focus is on designing an automated estimation procedure to identify linkage errors that may arise during the data linkage process. Specifically, this procedure is constructed to evaluate two key aspects: sensitivity, defined as the likelihood of correctly linking records belonging to the same individual, and positive predictive value (PPV), defined as the likelihood that linked records indeed belong to the same individual. The resulting validation algorithm can be applied to any dataset and any chosen linkage procedure, as long as the subset of records referred to are a gold standard (i.e., a set of records containing accurate identifiers, obtainable through processes such as manual matching).
In the second step, the objective is to develop a methodology for assessing the level of confidence in our research findings. This methodology considers the estimates of linkage errors (sensitivity and PPV) derived from the initial step to calculate the potential deviations of findings from the true values, as well as the degree of variability in estimations.
The utilization of large-scale administrative data enables the direct examination of nationwide health policy impacts while bypassing the need for data interpolations. When records lack distinctive identifiers, record linkage techniques could be used to create proxy identifiers that enable longitudinal patient-level analyses. However, issues with data quality can significantly affect linkage accuracy, and the presence of linkage errors is likely to sway and distort the findings’ true effects. Along with the NHLS in South Africa, the validation algorithm accounts for linkage errors when interpreting findings. This collaborative effort enhances the robustness of record linkage, ultimately enhancing the reliability of insights drawn from administrative health data.
*
Learn more about the Summer in the Field Fellowship Program.