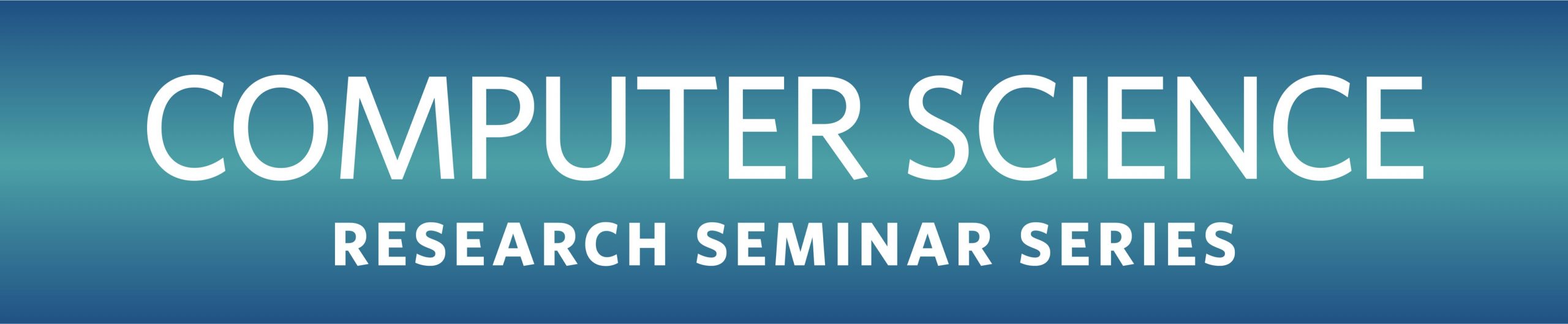
Computer Science Research Seminar Series
Presented by MET Department of Computer Science, this series features a 30 minute virtual talk followed by Q&A. All BU students and faculty are welcome to attend.
Upcoming Seminars
Making AI Impactful in Healthcare | Dr. Soroush Saghafian
Making AI Impactful in Healthcare
Guest Speaker: Dr. Soroush Saghafian
Moderated by Dr. Reza Rawassizadeh, Assistant Prof of CS
This virtual event will be held on-
Monday, 14th April, 2025 at 10:00 am EST
Abstract: There is increasing evidence that Machine Learning and Artificial intelligence algorithms can be used to enhance clinical care. In this talk, I address two critical aspects that can significantly improve the impact of such algorithms in healthcare practices: (1) moving beyond associations and creating algorithms capable of causal reasoning under ambiguity, and (2) a human-algorithm “centaur” model of care and decision-making, in which the power of human intuition is combined with the outstanding capabilities of algorithms. I describe our latest research on these subjects at the Public Impact Analytics Science Lab (PIAS-Lab) at Harvard, and discuss findings based on our various collaborations with the Mayo Clinic, Mass General Hospital, and some other public and private organizations.
Bio: Soroush Saghafian is an Associate Professor at Harvard University. He is the founder and director of the Public Impact Analytics Science Lab (PIAS-Lab) at Harvard, which is devoted to advancing and applying the science of analytics to solving societal problems that can have a public impact. He also serves as a core faculty member for the Harvard Center for Health Decision Science, a faculty affiliate for (a) the Harvard Data Science Initiative, (b) Harvard Ph.D. Program in Health Policy, and (c) the Harvard Mossavar-Rahmani Center for Business and Government, and is an associate faculty member at the Harvard Ariadne Labs (a pioneer lab in health systems innovation). He is an expert in healthcare analytics and healthcare operations management and has been collaborating with a variety of hospitals to improve their operational efficiency, patient flow, medical decision-making, and more broadly, healthcare delivery policies. Dr. Saghafian’s research has appeared in the news, including in pieces and interviews by PBS News, NBC News, Fast Company, Euronews, The Hill, National Academy of Medicine, Harvard Crimson, New-Meical.net, Industry Global News 24, Global Health News Wire, Eureka Alert (American Association for the Advancement of Science), Medscape, Medical Xpress, Managed Healthcare Executive, DOTmed, ABC Channel (TV), Devdiscourse.com, and INFORMS. He has won various awards for his research, including the INFORMS MSOM Young Scholar Prize for “outstanding contributions to scholarship in operations management,” INFORMS MSOM Responsible Research Award (second place) for “contributing knowledge that may have implications for making the world a better place,” the Inaugural INFORMS Mehrotra Research Excellence Award “for significant contributions to the practice of health applications through operations research and management science modeling and methodologies,” INFORMS Pierskalla Award “for the best research paper in healthcare,” and the Production and Operations Management Society (POMS) College of Healthcare Best Paper Award. He serves on the editorial board of a few journals, including Management Science, Operations Research, POM, Service Science, and IISE Transactions [Full CV here].
Seminar Archive
Fantastic Photonic Computing Systems and How to Use Them for AI | Dr. Ajay Joshi
Fantastic Photonic Computing Systems and How to Use Them for AI
Guest Speaker: Dr. Ajay Joshi, Professor, Department of ECE, BU
Moderated by Dr. Avinash Mohan, Assistant Prof of CS
Friday, 28th March, 2025
Abstract: Artificial Intelligence (AI)-based computing is commonly used today in a variety of domains including finance, healthcare, supply chain, and education. To support the ever-growing demands of AI-based computing workloads, there is a recent resurgence in using photonics for computing. However, photonics is not a panacea and should be used only where appropriate. In this talk, I’ll present three different electro-photonic AI accelerator designs – ADEPT, MIRAGE, and PhotoHDC, that we have developed in our group. ADEPT focuses on efficient DNN inference. It leverages a photonic computing core for efficiently performing GEMM operations, a vectorized digital electronic ASIC for performing non-GEMM operations, and SRAM arrays for storing DNN parameters and activations. MIRAGE builds on ADEPT and accelerates DNN training. By combining Residue Number System (RNS) and photonics, MIRAGE provides high energy efficiency without compromising precision and can successfully train state-of-the-art DNNs achieving accuracy comparable to FP32 training. PhotoHDC uses a combination of hyperdimensional computing (HDC) and photonics, two technologies that complement each other very well. PhotoHDC achieves multiple orders of magnitude energy-efficient HDC training and inference as compared to prior art.
Bio: Ajay Joshi received his Ph.D. degree from Georgia Tech in 2006 and then worked as a postdoctoral researcher at MIT. In 2009, he joined the ECE department at Boston University, where he is currently a Professor. He was a Visiting Researcher at Google in 2017-18 and an Architect at Lightmatter Inc. He recently co-founded a company, CipherSonic Labs, which is focused on data privacy. His research is in the areas of computer architecture and digital VLSI with focus on data security and privacy, machine learning and photonic computing. He received the NSF CAREER Award in 2012, Boston University ECE Department’s Award for Excellence in Teaching in 2014, Best Paper Award at ASIACCS 2018 and HOST 2023, and Google Faculty Research Award in 2018 and 2019. He currently serves as the Associate Editor for IEEE Transactions on VLSI Systems.
Understanding LLMs: How They Function and How They Have Changed | Dr. Sebastian Ratschka
Understanding LLMs: How They Function and How They Have Changed
Guest Speaker: Dr. Sebastian Ratschka, Staff Research Engineer at Lightning AI
Moderated by Dr. Reza Rawassizadeh, Associate Prof of CS
Abstract: This talk will guide the audience through the key stages of developing large language models (LLMs). We’ll start by explaining how these models are built, including the coding of their architectures. Next, we’ll discuss the processes of pretraining and fine-tuning, detailing what these stages involve and why they are important. In this talk, attendees will also learn about recent developments, including how model architectures have evolved from early GPT models to Llama 3.1. Additionally, the talk will provide an overview of the most recent training recipes, which include multi-stage pre-training and post-training.
Bio: Sebastian Raschka, PhD has been working on machine learning and AI for more than a decade. Sebastian joined Lightning AI in 2022, where he is a Staff Research Engineer focusing on AI and LLM research and development. Prior to that, Sebastian worked at the University of Wisconsin-Madison as an assistant professor in the Department of Statistics, focusing on deep learning and machine learning research. He has a strong passion for education and is best known for his bestselling books on machine learning using open-source software. You can find out more about Sebastian’s current projects at https://sebastianraschka.com/.
Combining the Power of Biobanks and System Biology Approaches for Better Infectious Disease Prevention and Treatment | Dr. Samira Asgari
Guest Speaker: Dr. Samira Asgari, Assistant Professor of Genetics and Genomic Sciences at the Icahn School of Medicine at Mount Sinai, NY
Moderated by Dr. Reza Rawassizadeh, Associate Prof of CS
Friday, November 15, 2024 at 10:00 AM EST
Abstract: The clinical outcome of any infectious disease can vary widely, ranging from asymptomatic cases to fatal outcomes, depending on a complex interplay between the pathogen, host, and environment. Traditionally, the study of infectious diseases has focused primarily on the pathogen, which has limited our understanding of the environmental and host-specific factors that influence disease susceptibility and severity. Addressing this gap is crucial for developing effective strategies and treatments for infectious disease control. In this talk, I will present our research on the role of human genetic factors and social determinants of health in infectious disease outcomes. I will also demonstrate how we can harness the power of large-scale biobanks and systems biology approaches to gain deeper insights into the complex mechanisms that determine the clinical outcomes of infectious diseases.
Bio: Dr. Asgari is an Assistant Professor of Genetics and Genomic Sciences at the Icahn School of Medicine at Mount Sinai, NY. She completed her M.Sc. in stem cell biology at the University of Tehran, Iran, her Ph.D. in human genomics of infectious diseases at the Lausanne Federal Institute of Technology, Switzerland, and her postdoctoral training in statistical genomics at Brigham and Women’s Hospital, USA. The overarching goal of the Asgari lab is to understand how human genetic, demographic, and environmental diversity translates to phenotypic diversity in the immune system and how these variations impact the clinical outcomes of infectious diseases. The lab achieves this goal by integrating electronic health records, multi-omics datasets, in vitro and in silico experimental models, statistical inference, and machine learning approaches.
Expert-Informed, User-Centric Explanations of Medical Image Classification | Dr. Michael Pazzani
Expert-Informed, User-Centric Explanations of Medical Image Classification
Guest Speaker: Dr. Michael Pazzani is the Director of the AI4Health Center and Research Director, Distinguished Principal Scientist, and Senior Supervising Computer Scientist of the Information Sciences Institute at the University of Southern California
Moderated by Dr. Reza Rawassizadeh, Associate Prof of CS
Friday, October 4, 2024 at 4:00 PM EST
Abstract: We argue that the dominant approach to explainable AI for explaining image classification with deep learning– annotating images with heatmaps, provides little value for users unfamiliar with deep learning. Instead, we argue that explainable AI for images should produce output like experts produce when communicating with one another, with apprentices, and with novices. We discuss a bit of the history of interpretable and explainable AI with examples from AI & medicine. A new approach that labels image regions with diagnostic features is proposed and evaluated. We draw on examples from radiology, ophthalmology, dermatology as well as bird classification.
Bio: Michael Pazzani is the director of the AI4Health Center at the University of Southern California. Dr Pazzani received his Ph.D. Computer Science, University of California, Los Angeles, and is a fellow of the Association for the Advancement of Artificial Intelligence. Dr. Pazzani started his career as an assistant, associate, and full professor of Information and Computer Science at the University of California, Irvine, and has served as the Director of the Information and Intelligent Systems Division at the National Science Foundation and as a member of the Board of Regents of the National Library of Medicine at the National Institutes of Health
A Unified Framework to Calculate Every Deep Learning Architecture by Hand | Dr. Tom Yeh
A Unified Framework to Calculate Every Deep Learning Architecture by Hand
Guest Speaker: Dr. Tom Yeh, Associate Professor in the Department of Computer Science and Principal Investigator at the Imagine AI Lab at the University of Colorado Boulder
Moderated by Dr. Reza Rawassizadeh, Associate Professor of CS and Eugene Pinsky, Associate Professor of the Practice, Computer Science; Coordinator, Software Development
Friday, September 13, 2024 at 10:00 AM EST
Abstract: I will present AI by Hand, a new framework I developed to demonstrate the core calculations of a wide range of deep learning architectures by hand, including basic building blocks such as artificial neuros and multi-layer perceptron, well-known architectures such as the transformer,, and state-of-art architectures such as the Diffusion Transformer and the Switch Transformer.
Bio: Prof. Tom Yeh leads the Imagine AI Lab at the University of Colorado Boulder. His research and teaching spans AI, HCI, Education, Ethics, and Neuroscience. He published more than 150 papers. He is the author of the popular AI by Hand ✍️ series, with by more than 70K followers across LinkedIn and X. At his university, Prof. Tom Yeh received several awards, including the Student Affair Faculty of the Year Award.
Using Everyday Routines as a Resource for Understanding Behaviors and Making Recommendations | Dr. Anind K. Dey
Using Everyday Routines as a Resource for Understanding Behaviors and Making Recommendations
Guest Speaker: Dr. Anind K. Dey, Professor and Dean of the Information School at the University of Washington.
Moderated by Dr. Reza Rawassizadeh, Associate Professor of Computer Science, Boston University Metropolitan College
Monday, April 15 at 2:30pm EST
Abstract: We live in a world where the promise of ubiquitous computing and the Internet of Things is coming true. We have smart devices that pervade our lives, and that are constantly collecting data about us and mostly discarded as irrelevant. I will demonstrate how researchers can extract relevance from this passively collected data and use it to “image” people’s behaviors. I will describe approaches for extracting behavioral routines from smart devices, and then how these routines can help us better understand individual and group human behaviors, as well as anomalies. Using examples from healthcare, I will describe how we can leverage both routines and anomalies to improve our understanding of health-related behaviors and make recommendations to support behavior change, including substance abuse, depression, and job performance.
Bio: Anind K. Dey is a Professor and Dean of the Information School at the University of Washington. Anind is renowned for his early work in context-aware computing, an important theme in modern computing, where computational processes are aware of the context in which they operate and can adapt appropriately to that context. His research is at the intersection of human-computer interaction, machine learning, and ubiquitous computing. For the past few years, Anind has focused on passively collecting large amounts of data about how people interact with their phones and the objects around them, to use for producing detection and classification models for human behaviors of interest. He applies a human-centered and problem-based approach through a collaboration with an amazing collection of domain experts in areas of substance abuse (alcohol, marijuana, opioids), mental health, driving and transportation needs, smart spaces, sustainability, and education. Anind was inducted into the ACM SIGCHI Academy for his significant contributions to the field of human-computer interaction in 2015 and was named an ACM Fellow in 2022. Before starting at the University of Washington in 2018, Anind was the Charles M. Geschke Professor and Director of the Human-Computer Interaction Institute at Carnegie Mellon University for 4 years, and was a member of the faculty for 13 years. Previously, he was a Senior Researcher at Intel Research and an Adjunct Assistant Professor of Computer Science at UC Berkeley. Anind received his PhD and MS in computer science, and an MS in aerospace engineering from Georgia Tech, and a Bachelors in Computer Engineering from Simon Fraser University.
Empowering Engagement: Data-Driven Strategies in Digital Health and Wellbeing | Dr. Uichin Lee
Empowering Engagement: Data-Driven Strategies in Digital Health and Wellbeing
Guest Speaker: Dr. Uichin Lee, Professor in the School of Computing, Korea Advanced Institute of Science and Technology (KAIST)
Moderated by Dr. Reza Rawassizadeh, Associate Professor of Computer Science, Boston University Metropolitan College
Friday, March 1 at 10:00 AM EST
Abstract: The use of mobile, wearable, and Internet of Things (IoT) technologies fosters unique opportunities for designing novel intelligent positive computing services that aim to realize human wellbeing and potential. These services can help with mental and social wellbeing (e.g., stress care and social-emotional learning), physical wellbeing (e.g., diet, exercise, and sleep coaching), and work productivity (e.g., attention management). This talk overviews the concept of data-driven digital health and wellbeing from the perspective of engagement empowerment by illustrating how sensor and interaction data collected from mobile, wearable, and IoT technologies are used to detect health and wellbeing issues, enable novel context-aware interventions, and analyze digital health and wellbeing services. Through critically reflecting on the literature and services, this talk discusses several research directions on empowering engagement, such as user and intervention modeling and management, as well as concerns and challenges, such as side effects, privacy, and ethical issues.
Bio: Dr. Uichin Lee is a Professor in the School of Computing at the Korea Advanced Institute of Science and Technology (KAIST), leading the Interactive Computing Lab, whose mission is to study intelligent positive computing systems that can intervene in threats to human health and digital wellbeing. He received a Ph.D. degree in computer science from UCLA in 2008. He worked for Alcatel-Lucent Bell Labs as a member of the technical staff before joining KAIST in 2010. He has joint affiliations with the Department of Industrial and Systems Engineering, the Graduate School of Data Science at KAIST, and the KAIST Health Science Institute. In 2023, he was inducted as a member of the SIGCHI Academy, an honorary group of individuals who have made substantial contributions to the field of human-computer interaction (HCI). He served as a program committee member of the key HCI conferences and journals, such as ACM CHI, CSCW, and Ubicomp, and as an editor for PACM HCI (CSCW) and IMWUT (Ubicomp). He received the best paper awards at ACM CHI’16, AAAI ICWSM’13, IEEE CCGrid’11, and IEEE PerCom’07, and an impact award from IEEE IoT Fourm’19.
Computational Behavior Modeling for Personalized Health | Dr. Afsaneh Doryab
Computational Behavior Modeling for Personalized Health
Guest Speaker: Dr. Afsaneh Doryab, Assistant Professor of Computer Science and Systems Engineering at the University of Virginia
Moderated by Dr. Reza Rawassizadeh, Associate Professor of Computer Science, Boston University Metropolitan College
Friday, February 2 at 10:00 AM EST
Abstract: Humans interact extensively with a wide range of computing devices, generating data streams that can be analyzed to extract cues about their physical and mental states. This latent information can be used to create more intelligent systems that can anticipate users’ needs and provide personalized services and interventions. However, this capability also poses new technical challenges. In this talk, I will present my research in modeling human behavior from multimodal data streams to address some of those challenges. I will showcase how we integrate behavior models into music melodies and social robots for personalized intervention.
Speaker Bio: Afsaneh Doryab is an Assistant Professor of Computer Science and Systems Engineering at the University of Virginia. Prior to joining UVA, she was a Systems Scientist at Carnegie Mellon University’s School of Computer Science. Her research lies at the intersection of Ubiquitous Computing, Artificial Intelligence, Human-Computer Interaction, and Digital Health. Dr. Doryab’s work revolves around creating computational models of human behavior using data streams collected through mobile, wearable, and embedded sensors. She applies her research to various domains, including health, social, and economic sectors. Her work has received funding from the National Science Foundation and the National Institutes of Health and has been published in top-tier conferences and journals such as IMWUT, CHI, CSCW, and JMIR.
SPLICE: Securing the lifecycle of Smart Homes | Dr. David Kotz
SPLICE: Securing the lifecycle of Smart Homes
Guest Speaker: Dr. David Kotz, the Provost, and Pat and John Rosenwald Professor in the Department of Computer Science at Dartmouth College
Moderated by Dr. Farshid Alizadeh-Shabdiz , Associate Professor of the Professional Practice, Boston University Metropolitan College
Friday, November 17 at 2:30 PM EST
Abstract: Our homes are increasingly embedded with “Smart Things,” networked with each other and with the Internet, forming “Smart Homes.” What happens when an occupant moves out or transfers ownership of her Smart Home? How does an occupant identify and decommission all the Things in a home before she moves out? How does a new occupant discover, identify, validate, and configure all the Things in the home he adopts? When a person moves from smart home to smart office to smart hotel, how is a new environment vetted for safety and security, how are personal settings migrated, and how are they securely deleted on departure? When the original vendor of a Thing (or the service behind it) disappears, how can that Thing (and its data, and its configuration) be transferred to a new service provider? What interface can enable lay people to manage these complex challenges, and be assured of their privacy, security, and safety? We present a list of key research questions to address these important challenges, then give an overview of results from our own collaborative research project, SPLICE: Security and Privacy in the Lifecycle of IoT in Consumer Environments.
Speaker Bio: David Kotz is the Provost, and the Pat and John Rosenwald Professor in the Department of Computer Science, at Dartmouth College. He previously served as Associate Dean of the Faculty for the Sciences, as a Core Director at the Center for Technology and Behavioral Health, and as the Executive Director of the Institute for Security Technology Studies. His current research involves security and privacy in smart homes, and wireless networks. He has published over 250 refereed papers, obtained $89m in grant funding, given over 200 invited lectures, and mentored over 100 research students and postdocs. He is an ACM Fellow, an IEEE Fellow, a 2008 Fulbright Fellow to India, a 2019 Visiting Professor at ETH Zürich, and an elected member of Phi Beta Kappa. He received his AB in Computer Science and Physics from Dartmouth in 1986, and his PhD in Computer Science from Duke University in 1991.
Machine Learning and the Data Center: A Dangerous Dead End | Dr. Nicholas D. Lane
Machine Learning and the Data Center: A Dangerous Dead End
Guest Speaker: Dr. Nicholas D. Lane, Professor of Computer Science and Technology at the University of Cambridge and a Fellow of St. John’s College
Moderated by Dr. Reza Rawassizadeh, Associate Professor of Computer Science, Boston University Metropolitan College
Abstract: The vast majority of machine learning (ML) occurs today in a data center. But there is a very real possibility that in the (near?) future, we will view this situation similarly to how we now view lead paint, fossil fuels and asbestos: a technological means to an end, that was used for a time because, at that stage, we did not have viable alternatives – and we did not fully appreciate the negative externalities that were being caused. Awareness of the unwanted side effects of the current ML data center centric paradigm is building. It couples to ML an alarming carbon footprint, a reliance to biased close-world datasets, serious risks to user privacy – and promotes centralized control by large organizations due to the assumed extreme compute resources. In this talk, I will offer a sketch of preliminary thoughts regarding how a data center free future for ML might come about, and also describe how some of our recent research results and system solutions (including the Flower framework — http://flower.dev) might offer a foundation along this path.
Speaker Bio: Nic Lane (http://niclane.org) is a full Professor in the department of Computer Science and Technology, and a Fellow of St. John’s College, at the University of Cambridge. He also leads the Cambridge Machine Learning Systems Lab (CaMLSys — http://http://mlsys.cst.cam.ac.uk/). Alongside his academic appointments, Nic is the co-founder and Chief Science Officer of Flower Labs (https://flower.dev/), a venture-backed AI company (YCW23) behind the Flower framework. Nic has received multiple best paper awards, including ACM/IEEE IPSN 2017 and two from ACM UbiComp (2012 and 2015). In 2018 and 2019, he (and his co-authors) received the ACM SenSys Test-of-Time award and ACM SIGMOBILE Test-of-Time award for pioneering research, performed during his PhD thesis, that devised machine learning algorithms used today on devices like smartphones. Nic was the 2020 ACM SIGMOBILE Rockstar award winner for his contributions to “the understanding of how resource-constrained mobile devices can robustly understand, reason and react to complex user behaviors and environments through new paradigms in learning algorithms and system design.
Foresight: A Game-Theory Hybrid Algorithm with Reinforcement-Learning for Predictive Analytics | Dr. Shahram Rahimi
Foresight: A Game-Theory Hybrid Algorithm with Reinforcement-Learning for Predictive Analytics
September 29, 2023
Guest Speaker: Dr. Shahram Rahimi, Gloria & Douglas Marchant Endowed Chair, Professor and Head of the Department of Computer Science and Engineering at Mississippi State University
Moderated by Dr. Reza Rawassizadeh, Associate Professor of Computer Science, Boston University Metropolitan College
Abstract: An innovative and forward-looking hybrid predictive analytics algorithm will be presented. Unlike traditional machine learning-based technologies, Foresight leverages the current dynamics rather than relying solely on historical data analysis to make accurate predictions. This algorithm incorporates game theory, expected utility and median vector theories, and reinforcement learning. One of the key differentiators of Foresight is its incorporation of reinforcement learning, which enables the modeling of players’ reasoning abilities in assessing risks. This mechanism enhances the algorithm’s predictive capabilities, particularly when applied to complex problems involving multiple stakeholders with conflicting interests in economic, business, or political issues. By accounting for these complexities, Foresight becomes a valuable tool in predicting outcomes and informing decision-making processes. To demonstrate the effectiveness of this algorithm, we will illustrate several compelling case studies, showcasing its accuracy and performance in real-world scenarios. These case studies will highlight the algorithm’s ability to anticipate outcomes in compound problems, displaying its reliability and practicality.
Speaker Bio: Dr. Shahram Rahimi holds the Gloria & Douglas Marchant Endowed Chair and serves as Professor and Head of the Department of Computer Science and Engineering at Mississippi State University. Before his current position, Dr. Rahimi led the Department of Computer Science at Southern Illinois University for five years. Dr. Rahimi is a recognized leader in artificial and computational intelligence with over 250 peer-reviewed articles and a few patents or pending patents in this area. His commitment to advancing the field extends beyond academia. Dr. Rahimi actively contributes to the IEEE New Standards Committee in Computational Intelligence and provides invaluable advice to the staff and administration at the state department on predictive analytics for foreign policy. In 2016, Dr. Rahimi was honored with the prestigious Illinois Rising Star award from ISBA. His groundbreaking intelligent algorithm for patient flow optimization and hospital staffing has been implemented in over 1000 emergency departments nationwide. Notably, it was named one of the top 10 AI technologies for healthcare in 2018 by HealthTech magazine. Throughout his career, Dr. Rahimi has secured more than $40 million in research funding as Principal Investigator (PI) or Co-PI from federal agencies and industry sources. His entrepreneurial spirit led him to co-found Potentia Analytics Inc. in 2013, a company dedicated to applying AI to Healthcare Management. Potentia Analytics has made substantial strides in the field, providing cutting-edge technology to over 20% of hospitals in the United States and globally. In addition, Dr. Rahimi has organized 15 international conferences or workshops focusing on computational intelligence and multi-agent systems in past two decades.
Multimodal Machine Learning and Human Centered Computing for Health and Wellbeing | Dr. Akane Sano
Multimodal Machine Learning and Human Centered Computing for Health and Wellbeing
April 21, 2023
Guest Speaker: Dr. Akane Sano, Assistant Professor of Electrical and Computer Engineering, Rice University
Moderated by Dr. Reza Rawassizadeh, Associate Professor of Computer Science, Boston University Metropolitan College
Abstract: What if we can design data driven + human centered personalized feedback loop systems for patients, clinical stakeholders, and healthy people for processing and modeling multimodal clinical and moment-to-moment data and managing and improving health?
First, I will introduce potential and challenges in designing such a system by combining multimodal measurements such as electric health records, fMRI, and clinical assessment with field measurements via mobile and remote sensing.
Second, I will introduce a series of studies, algorithms, and systems we have developed for addressing these issues and measuring, predicting, and supporting personalized health and wellbeing.
More specifically, I will talk about (1) leveraging unlabeled data to design robust but interpretable models, (2) approaches that positively transfer knowledge from multiple modalities to fewer modalities in model deployment in the real world, and (3) balancing bias and performance in health prediction machine learning models and collecting diverse data samples in mobile health systems.
I will also discuss learned lessons and potential future directions in health and wellbeing research.
Speaker Bio: Dr. Akane Sano is an Assistant Professor at Rice University, Department of Electrical Computer Engineering, Computer Science, and Bioengineering. She directs the Computational Wellbeing Group and is a member of Rice Digital Health Initiative. Her research includes data science, machine learning, and human-centered intelligent systems for health and wellbeing and spans in the field of affective computing, ubiquitous and wearable computing, and biobehavioral sensing and analysis/modeling. She has been developing tools, algorithms, and systems to measure, forecast, understand and improve health and wellbeing using multimodal data from mobile and wearable devices in daily life settings, and clinical assessment. She received her Ph.D. at the Massachusetts Institute of Technology and her MEng and BEng at Keio University, Japan. Her recent awards include the NSF Career Award, the Best of IEEE Transactions on Affective Computing 2021, the Best Paper Award at IEEE BHI 2019 conference
Data-Efficient Deep Learning using Physics-Informed Neural Networks | Dr. Maziar Raissi
Data-Efficient Deep Learning using Physics-Informed Neural Networks
Guest Speaker: Dr. Maziar Raissi, Assistant Professor of Applied Mathematics, University of Colorado Boulder
Moderated by Dr. Reza Rawassizadeh, Associate Professor of Computer Science, Boston University Metropolitan College
Mar 24, 2023
Abstract: A grand challenge with great opportunities is to develop a coherent framework that enables blending conservation laws, physical principles, and/or phenomenological behaviours expressed by differential equations with the vast data sets available in many fields of engineering, science, and technology. At the intersection of probabilistic machine learning, deep learning, and scientific computations, this work is pursuing the overall vision to establish promising new directions for harnessing the long-standing developments of classical methods in applied mathematics and mathematical physics to design learning machines with the ability to operate in complex domains without requiring large quantities of data. To materialize this vision, this work is exploring two complementary directions:
(1) designing data-efficient learning machines capable of leveraging the underlying laws of physics, expressed by time dependent and non-linear differential equations, to extract patterns from high-dimensional data generated from experiments, and
(2) designing novel numerical algorithms that can seamlessly blend equations and noisy multi-fidelity data, infer latent quantities of interest (e.g., the solution to a differential equation), and naturally quantify uncertainty in computations.
Guest Speaker Bio: I am currently an Assistant Professor of Applied Mathematics at the University of Colorado Boulder (CU Boulder). I received my Ph.D. in Applied Mathematics & Statistics, and Scientific Computations from University of Maryland College Park. I then moved to Brown University to carry out my postdoctoral research in the Division of Applied Mathematics. I then worked at NVIDIA in Silicon Valley for a little more than one year as a Senior Software Engineer before moving to Boulder. My expertise lies at the intersection of Probabilistic Machine Learning, Deep Learning, and Data Driven Scientific Computing. I have been actively involved in the design of learning machines that leverage the underlying physical laws and/or governing equations to extract patterns from high-dimensional data generated from experiments.
Systems Research Towards Solving Societal Problems | Dr. Aruna Balasubramanian
Systems Research Towards Solving Societal Problems
February 27, 2023
Abstract: Much of systems and networking research focuses on improving performance, often defined in terms of throughput, latency, and robustness. Our lab have been focusing on systems research that also has societal impact. I will describe two such research threads: one on sustainable NLP and the other on accessibility
In the first part of the talk, I will describe systems optimizations we have developed that significantly reduce the compute, memory, and energy requirement of large NLP models. The optimizations we developed can be applied broadly and results in over 10x reduction in energy. Beyond optimizations, we have been working on accurate energy prediction of large NLP models. Existing energy prediction approaches are not accurate, making it difficult for developers and practitioners to reason about their models in terms of energy. I will describe our work on developing an accurate and interpretable energy model for NLP applications.
In the second part of the talk, I will describe our work on improving the accessibility of smartphone applications for users with disabilities. Blind users face several challenges when interacting with applications starting from fast-draining battery to having to use difficult touchscreen gestures. I will describe our work that builds on virtualization techniques to make smartphone interactions significantly more accessible.
Speaker Bio: Aruna Balasubramanian is an Associate Professor at Stony Brook University. She received her Ph.D from the University of Massachusetts Amherst, where her dissertation won the UMass outstanding dissertation award and was the Sigcomm dissertation award runner up. She works in the area of networked systems. Her current work consists of three threads: (1) significantly improving Quality of Experience of Internet applications, and (2) improving the usability, accessibility, and privacy of mobile systems, and (3) sustainable NLP.
She is the recipient of the SIGMobile Rockstar award, a Ubicomp best paper award, a Computing Innovation Fellowship, a VMWare Early Career award, several Google research awards, and the Applied Networking Research Prize. She is passionate about improving the diversity in Computer Science and leads the diversity committee in the department, is the faculty advisor for the WiCS and WPhD groups at Stony Brook, and is an active member of the N2Women group.
MaRz: Machine Learning on the Fly | Dr. Eric Braude
MaRz: Machine Learning on the Fly
January 9, 2023
Abstract: This research concerns machine learning on the fly, for problems where learning data changes continually, or where waiting for the system to learn is impractical. The principle behind MaRz (Machine Learning in Real Time by Fuzzification) is a fuzzy interpretation of data. This is a quantitative expression of the fact that each datum need not be taken literally. For example, a particular student with SAT score 1300 and college GPA of 3.5, among hundreds of thousands, can be just as appropriately thought of as having approximately 1300 and 3.5 scores respectively. MaRz expresses this via fuzzy values centered on traditional “crisp” values. We show results on increasingly complex data sets and discuss the technical issues involved.
Speaker Bio: Eric Braude has a Ph.D. in mathematics from Columbia University and master’s degree in computer science. He taught at CUNY and Penn State, followed by 12 years in government and industry as a software engineer, scientist, and manager. He is an associate professor of computer science at Boston University’s Metropolitan College. Eric has written, cowritten, or edited six books. His research concerns machine learning and program design.
Securing the Next Billion Consumer Devices on the Edge | Dr. Hamed Haddadi
Securing the Next Billion Consumer Devices on the Edge
December 9, 2022
Guest Speaker: Dr. Hamed Haddadi, Reader in Human-Centered Systems, Department of Computing, Imperial College London
Moderated by: Dr. Reza Rawassizadeh, Associate Professor of Computer Science
Abstract: In the next 5 years, I aim to address a major challenge in the adoption of user-centered privacy-enhancing technologies: Can we leverage novel architectures to provide private, trusted, personalized, and dynamically- configurable models on consumer devices to cater for heterogenous environments and user requirements? Importantly, such properties must provide assurances for the data integrity and model authenticity/trustworthiness, while respecting the privacy of the individuals taking part in training and improving such models , in particular when dealing with sensitive models and data from the consumer Internet of Things (IoT) devices. I will discuss some of the use cases in the space of IoT, edge computing, and browsers.
Speaker Bio: Hamed is a Reader in Human-Centered Systems at the Department of Computing at Imperial College London. He also serves as a Security Science Fellow of the Institute for Security Science and Technology. In his industrial role, he is the Chief Scientist at Brave Software where he works on developing privacy-preserving analytics protocols. He is interested in User-Centered Systems, IoT, Applied Machine Learning, and Data Security & Privacy. He enjoys designing and building systems that enable better use of our digital footprint, while respecting users’ privacy.
Dual-purpose Interaction: Designing interactive systems interwoven with what people already do | Dr. Koji Yatani
Dual-purpose Interaction: Designing interactive systems interwoven with what people already do
November 15, 2022
Guest Speaker: Dr. Koji Yatani, Associate Professor, School of Engineering, University of Tokyo
Moderated by Dr. Reza Rawassizadeh, Associate Professor of Computer Science
Abstract: Interweaving technology into our daily life is a dream of ubiquitous computing. Such integration not only achieves indistinguishability of technology, but also creates additional values and benefits in existing user interaction. In this talk, I am sharing our recent work that adds new benefits to existing user interaction and behavior. I present three projects that exploit existing user interaction in usable security and interactive machine teaching. I also share our envision on how HCI research can enrich people’s life through interface design with multiple purposes.
Speaker Bio: Dr. Koji Yatani (https://yatani.jp) is an Associate Professor and 2017 UTokyo Excellent Young Researcher in Department of Electrical Engineering and Information Systems (EEIS), School of Engineering at The University of Tokyo, where he leads Interactive Intelligent Systems Laboratory (https://iis-lab.org). He is also affiliated with Emerging Design and Informatics Course, Interfaculty Initiative in Information Studies. His research emphasizes on development and evaluations of IoT applications with novel sensing technology, productivity/creativity support, creative use of AI technology for interactive systems, and usable security. He was the winner of RIEC Award (2019); IPSJ/ACM Award for Early Career Contributions to Global Research (2020); Japan ACM SIGCHI Local Chapter Distinguished Young Researcher Award (2021); Funai Academic Award (2021); and IPSJ Microsoft Faculty Award (2022). He also received 2 Best Paper awards at CHI (2011 and 2016) as well as 3 Honorable Mention Awards at CHI (2014, 2020) and 2 at MobileHCI (2014). He was also a winner of School of Engineering Best Teaching Award at University of Tokyo (2022). Dr. Koji Yatani served as a founding editor for Proceedings of ACM Interactive, Mobile, Wearable and Ubiquitous Technology (2016–2020), a Papers co-Chair for CHI 2022, a Program co-Chair for UbiComp 2015, and a subcommittee co-chair for CHI 2019 and 2020. He was also a Steering Committee chair for UbiComp (2019 — 2021). He currently serves as a Vice chair for Japan ACM SIGCHI Chapter.
The Internet of Materials: Rethinking the future of computing | Dr. Gregory D. Abowd
The Internet of Materials: Rethinking the future of computing
October 3,2022
Guest Speaker: Dr. Gregory D. Abowd, Dean of the College of Engineering, Northeastern University
Moderated by Dr. Reza Rawassizadeh, Associate Professor of Computer Science
Abstract: If we trace how computers have evolved over the past 8 or so decades, we can certainly see the impact of increasingly sophisticated manufacturing techniques. Computers now come in many different shapes and sizes. And applications, of course, have driven the widespread adoption, so much so that it appears we have an insatiable appetite for computing, and the power that is needed to feed it. That’s a problem. We must take more seriously some of the past assumptions of how we manufacture computers and what properties the constituent materials impose. In this talk, I will introduce the notion of the Internet of Materials, whereby the power, form factor, and manufacturing costs of a computational object take precedence over other functional features of that object. I will show some simple examples that highlight how we can create self-sustaining computational materials. The purpose of the talk is to motivate researchers to think creatively about the convergence of materials, manufacturing, and computing. I hope these initial, and somewhat simple, examples prompt deeper discussions on how Northeastern can become a leader in defining a complementary computing industry.
Speaker Bio: Gregory D. Abowd is the Dean of the College of Engineering at Northeastern University, where he is also a Professor of Electrical and Computer Engineering with affiliate appointments in the Khoury College of Computer Sciences and the Bouvé College of Health Sciences. Prior to joining Northeastern in March 2021, Dr. Abowd was faculty in the College of Computing at the Georgia Institute of Technology for over 26 years, where he held the titles of Regents’ Professor and J.Z. Liang Endowed Chair in the School in Interactive Computing. His research falls largely in the area of Human-Computer Interaction with an emphasis on applications and technology development for mobile and ubiquitous computing in everyday settings. His research has introduced innovations in the classroom, the home, for stakeholders connected with autism, and sustainable forms of computing in everyday life. He has been the founding Editor-in-Chief for two major journals and is the most highly cited researcher in HCI and ubiquitous computing in the world, according to csrankings.org (the second two are both his former PhD students). Dr.
Abowd is a Fellow of the ACM and an elected member of the ACM SIGCHI Academy. He was a 2009 recipient of the ACM Eugene Lawler Humanitarian Contributions within Computer Science and Informatics. He earned his Bachelor of Science in Honors Mathematics (summa cum laude) from the University of Notre Dame in 1986 as well as a Master of Science (1987) and Doctor of Philosophy (1991) in Computation from the University of Oxford, where he attended as a Rhodes Scholar.
Ultra-low-power Acoustic Perception in IoT | Dr. Nirupam Roy
Ultra-low-power Acoustic Perception in IoT
April 8, 2022
Guest Speaker: Dr. Nirupam Roy, Assistant Professor in Computer Science, University of Maryland, College Park
Moderated by Dr. Reza Rawassizadeh, Associate Professor of Computer Science
Abstract: Agile and miniature robots are emerging with new capabilities and sensing skills. Insect-sized robots are becoming new first responders to search for survivors in disaster debris. Tiny drones, each a couple of inches in size, are helping mapping a hazardous location before deploying human rescuers. These small robotic systems, when paired with autonomous navigation, can create new possibilities in a wide range of crucial applications, including precision farming, disaster management, and surveillance and monitoring. However, it will require overcoming a set of challenges to realize the vision and perception of the environment probably the most crucial of them. It is, however, challenging for small robotic systems due to the unique constraints of limited energy source, low weight carrying capacity and size, small computational power, and the requirement of low-cost manufacturing (SWaP-C constraints). This talk presents recent works in ultra-low-power acoustic sensing and perception as an opportunity to bridge this gap.
Speaker Bio: Nirupam Roy is an Assistant Professor in Computer Science at the University of Maryland, College Park (UMD). He received his Ph.D. from the University of Illinois, Urbana-Champaign (UIUC) in 2018. His research interests are in wireless networking, mobile computing, and embedded systems with applications to IoT, cyber-physical systems, and security. His doctoral thesis was selected for the 2019 CSL Ph.D. thesis award at UIUC. Prof. Roy is the recipient of the Valkenburg graduate research award, the Lalit Bahl fellowship, and the outstanding thesis awards from both his Bachelor’s and Master’s institutes. His research received the MobiSys best paper award and was selected for the ACM SIGMOBILE research highlights. Many of his research projects have been featured in news media such as the MIT Technology Review, The Telegraph, and The Huffington Post.
Dense and Cyclic Gray Codes | Dr. Thomas Cormen
Dense and Cyclic Gray Codes
March 3, 2022
Guest Speaker: Dr. Thomas H. Cormen, Emeritus Professor, Dartmouth College
Moderated by Dr. Reza Rawassizadeh, Associate Professor of Computer Science
Abstract: The binary reflected Gray code, patented by Frank Gray in 1953, is a permutation of the sequence ⟨0, 1, …, n-1⟩, where n is a power of 2, with the property that the binary representation of each number in the sequence differs from that of the preceding number in exactly one bit. The binary reflected Gray code is also cyclic, in that the last and first numbers also differ in only one bit.
What if n is not a power of 2? How can we create a dense Gray code: a permutation of ⟨0, 1, …, n-1⟩ with the Gray-code property for any integer n > 1? The answer turns out to be surprisingly simple, but not obvious at first why it should work. When can we create a dense Gray code with the cyclic Gray-code property, and how do we do it? The answer here is a bit more obvious—once you have already seen it.
Time-permitting, I will also touch on Gray codes for radices other than 2 and for mixed radices. All results are joint work with my senior thesis students, Jessica Fan ’17 and Devina Kumar ’18.
Speaker Bio: Thomas H. Cormen is Emeritus Professor in the Dartmouth College Department of Computer Science, where he has been since 1992. He served as the department chair from 2009 to 2015, and he directed the Dartmouth Institute for Writing and Rhetoric from 2004 to 2008. Professor Cormen received the B.S.E. degree in Electrical Engineering and Computer Science from Princeton University in 1978 and the S.M. and Ph.D. degrees in Electrical Engineering and Computer Science from the Massachusetts Institute of Technology in 1986 and 1992, respectively. An ACM Distinguished Educator, he is coauthor of the leading textbook on computer algorithms, Introduction to Algorithms, which he wrote with Charles E. Leiserson, Ronald L. Rivest, and Clifford Stein. He is also the author of Algorithms Unlocked. Professor Cormen’s primary research interests are in algorithm engineering and parallel computing. He focuses on algorithms and software infrastructure to mitigate the high latency inherent in accessing the outer levels of the memory hierarchy and in interprocessor communication. Lately, he has also been examining fundamental questions about Gray codes.
Integrating Interactive Devices with the User's Body | Dr. Pedro Lopes
Integrating Interactive Devices with the User’s Body
February 1, 2022
Guest Speaker: Pedro Lopes, Assistant Professor in Computer Science, University of Chicago
Moderated by Dr. Reza Rawassizadeh, Associate Professor of Computer Science
Abstract: The main question that drives my research is: what is the next interface paradigm that supersedes wearable devices? I argue that the new paradigm is one in which interactive devices will integrate with the user’s biological senses and actuators. This way of engineering devices that intentionally borrow parts of the user’s biology puts forward a new generation of miniaturized devices; allowing us to circumvent traditional physical constraints. For instance, in the case of our devices based on electrical muscle stimulation, they demonstrate how our body-device integration circumvents the constraints imposed by the ratio of electrical power and size of a motor. Taking this further, we demonstrate how our body-device integration approach allowed us to also miniaturize thermal feedback (hot/cold sensations) without the need for power-hungry thermal devices. We believe that these bodily-integrated devices are the natural succession to wearable interfaces and allow us to investigate how interfaces might connect to our bodies in a more direct and personal way.
Speaker Bio: Pedro Lopes is an Assistant Professor in Computer Science at the University of Chicago. Pedro focuses on integrating computer interfaces with the human body—exploring the interface paradigm that supersedes wearable computing. Some of these new integrated-devices include: muscle stimulation wearable that allows users to manipulate tools they have never seen before or that accelerate their reaction time, or a device that leverages the sense of smell to create an illusion of temperature. Pedro’s work has received a number of academic awards, such as four ACM CHI/UIST Best Papers. It also captured the interest of the media, such as New York Times or Wired and was exhibited at Ars Electronica & World Economic Forum. (More: https://lab.plopes.org)
Developing a Framework for Natural Language Processing of Clinical Documents | Dr. Frank Meng
Developing a Framework for Natural Language Processing of Clinical Documents
January 25, 2022
Guest Speaker: Dr. Frank Meng, Assistant Professor of Medicine in General Internal Medicine, Boston University School of Medicine
Moderated by Dr. Guanglan Zhang, Associate Professor of Computer Science
Abstract: The capturing of increasingly more and more patient data by healthcare systems has enabled much innovative advancements in medical science and patient care through the application of artificial intelligence (AI) and other data-driven technologies. However, much useful information continues to be locked within free text documents, limiting the usefulness of the data to the elements that are already being captured in structured format. In this talk, I will present use cases for implementing natural language processing (NLP) systems that automate the extraction of information from clinical documents with the goal of illustrating the unique challenges of working within the healthcare domain. In addition, I will also provide an overview of the Department of Veterans Affairs (VA) Healthcare System and the VA’s large-scale patient data repository.
Speaker Bio: Frank Meng, PhD is an Assistant Professor of Medicine in General Internal Medicine at the Boston University School of Medicine and the Associate Director of Clinical Informatics at the VA Boston Healthcare System. Before entering academia, Dr. Meng worked as a software engineer and a project leader at companies like IBM and The Aerospace Corporation. He began working in the medical field in 2013 when he first taught a course called “Introduction to medical informatics seminar” at UCLA. Since then he has published papers and given lectures, but his technological background is apparent in his writing, which includes big data and informatics as they related to the medical field.
How to Make Your Own StatQuest!!! | Dr. Josh Starmer
How to Make Your Own StatQuest!!!
December 6, 2021
Guest Speaker: Dr. Josh Starmer, StatQuest
Moderated by Dr. Reza Rawassizadeh, Associate Professor of Computer Science
Abstract: Hi! I’m Josh Starmer and I make YouTube videos that clearly explain Data Science, Statistics and Machine Learning Topics. In this talk I’ll describe the methods I use to research and master new material as well as the tips, tricks and design principles that I use to create presentations that allow the audience to focus on the main ideas.
Speaker Bio: Josh Starmer is the person behind the popular YouTube channel, “StatQuest with Josh Starmer.” Since 2016, Josh has used an innovative and unique visual style to clearly explain Statistics, Data Science and Machine Learning concepts and algorithms to curious people all over the world. Rather than dumb down the material, Josh brings people up with simple examples worked through, step-by-step, using pictures to make sure every main idea is easy to understand and remember. Josh is called the “Patron Saint of Silicon Valley” because people binge watch StatQuest videos before job interviews, the “Bill Nye of Statistics” by making the topic fun and exciting and the “Bob Ross of Data” by cutting through the hype and helping people relax with silly songs.
Extrapolating the Research Timeline of HCI: Cyber Meets Physical |Dr. Max Mühlhäuser
Extrapolating the Research Timeline of HCI: Cyber Meets Physical
November 17, 2021
Speaker:Dr. Max Mühlhäuser, Professor, University of Darmstadt
Moderated by Dr. Reza Rawassizadeh, Associate Professor of Computer Science
Abstract:We witness the era of digital transformation: ever more areas of our business and everyday lives are pervaded by information and communication technologies. However, the majority of *interactions* between humans and this “new digital world” still happens through traditional computer-based means. This implies interaction via screens, which by their very nature *separate* the physical world—and thus people—from the digital world.
In order to overcome this barrier, two ‘movements’ must be pursued in HCI research and development: in one direction, the digital world and corresponding interaction must become tightly integrated with the physical world and with the way we perceive and interact with it; in the other direction, physical objects must become ‘naturally’ digitally interactive in the sense of Human Cyber-Physical Interaction (“HCI” revisited).
The talk will first explain the interaction obstacle mentioned in the first paragraph from a historic perspective of HCI. Thereafter, the two required grand directions of HCI R&D will be discussed. A number of recent research advancements will be mentioned as examples, and showcased via video. The talk will end with a (not so serious) sneak preview into the newest interaction modality.
Speaker Bio: Max Mühlhäuser is a full professor at Technical University of Darmstadt and head of the Telecooperation Lab. He holds key positions in several large collaborative research centers and is leading the Doctoral School on Privacy and Trust for Mobile Users. He and his lab members conduct research on Human Computer Interaction, the future Internet, Intelligent Systems, and Cybersecurity including Privacy & Trust. Max founded and managed industrial research centers, and worked as either professor or visiting professor at universities in Germany, the US, Canada, Australia, France, and Austria. He is a member of acatech, the German Academy of the Technical Sciences. He was and is active in numerous conference program committees, as organizer of several annual conferences, and as a member of editorial boards or a Guest Editor for journals such as ACM IMWUT, ACM ToIT, Pervasive Computing, ACM Multimedia, and Pervasive and Mobile Computing.
Vulnerability Relationship between Feature Vector Scale & Convolutional Neural Networks w/ Adversarial Examples | Dr. Sang-Woong Lee
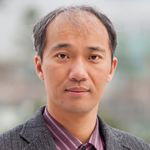
October 21, 2021
Guest Speaker: Dr. Sang-Woong Lee, Professor, Gachon University
Moderated by Dr. Reza Rawassizadeh, Associate Professor of Computer Science
Abstract: In the field of image classification, Deep Convolutional Neural Networks can misclassify images by perturbation noise. Images that are artificially created to cause misclassification are called adversarial examples. There are various conjectures about why DCNN is vulnerable to noise such as adversarial examples. We hypothesize that DCNN is vulnerable to noise because of ‘unfair data learning’. Furthermore, we assume that ‘unfair data learning’ learns the scale of feature vectors differently in feature spaces. Thus, the trained data will exhibit different vulnerabilities against noise depending on the scale of the feature vector. We use DCNN and CIFAR-10 datasets to conduct vulnerability tests for each scale section of feature vectors. Vulnerability experiments compare cosine similarity from each feature vector of the original and noisy images and observe the error rate by scale sections. Experimental results showed sensitive results with low cosine similarity and a high error rate in the small-scale section. On the other hand, in the high-scale section, the result shows robustness for noise with high cosine similarity and low error rate.
Speaker Bio: Sang-Woong Lee received the B.S. degree in electronics and computer engineering and the M.S. and Ph.D. degrees in computer science and engineering from Korea University, Seoul, South Korea, in 1996, 2001, and 2006, respectively. From June 2006 to May 2007, he was a Visiting Scholar with the Robotics Institute, Carnegie Mellon University. From September 2007 to February 2017, he was a Professor with the Department of Computer Engineering, Chosun University, Gwangju, South Korea. He is currently a Professor with the Department of Software, Gachon University. His current research interests include face recognition, computational aesthetics, machine learning, and medical imaging analysis.
Passive Sensing Analytics and Mobile Health | Dr. Nabil Alshurafa
Passive Sensing Analytics and Mobile Health
September 23, 2021
Speaker: Dr. Nabil Alshurafa, Assistant Professor of Preventive Medicine, Northwestern University
Moderated by Dr. Reza Rawassizadeh, Associate Professor of Computer Science
Abstract: Researchers seek to understand human behaviors in their natural setting so they can design interventions that help manage symptoms, prevent illness, and improve health and wellbeing. Wearables (with embedded sensors) combined with machine learning algorithms are increasingly being adopted to understand human behavior. Through analysis of continuous streams of data provided by these sensors, machine learning and analytics pipelines are used to understand a person’s moment-to-moment behavior, psychological state and environmental contexts in which the behavior occurs. This is allowing researchers to understand the interplay between behavior, physiological states and environmental influences along with individual’s physical and mental health. One important goal is to be able to use these novel methods to detect and predict appropriate times to apply interventions that improve health and well-being.
In this talk I hope to go through an overview of the end-to-end process needed for analyzing passive sensing data and inferring human behavior using wearables (with examples in eating and stress). We will go through an example passive sensing data analytic chain (PASDAC), which enables users to clean, curate, segment, classify and evaluate the signals generated from wearable sensors using signal processing and machine learning. We will also touch on current challenges and opportunities for research at the intersection of passive sensing data analytics and mobile health.
Speaker Bio: Nabil Alshurafa is an Assistant Professor of Preventive Medicine and of Computer Science (courtesy) and Electrical and Computer Engineering (courtesy) at Northwestern University. He received his Ph.D. in Computer Science at the University of California Los Angeles (UCLA) in 2015, where his dissertation was awarded the Computer Science outstanding graduating student award, and the Symantec outstanding research award. In 2015, Popular Science magazine highlighted his research on designing a wearable neck-worn sensor WearSens to distinguish between solid and liquid foods consumed. He currently leads the Sensor Analytics program in the Institute for Augmented Intelligence in Medicine and directs the HABits Lab at Northwestern, which aims to bridge between computer science and behavioral science research. His current research seeks to transform our understanding of health constructs by designing objective verifiable wearable sensor measures and machine learning analytic pipelines, to more effectively design interventions that improve lifestyle habits. In 2018, he was awarded a five-year NIDDK NIH Career award, to develop expertise in obesity-related research and advance passive sensing of problematic eating behaviors. He was awarded an NSF EAGER interdisciplinary award, to build privacy conscious technology and analytics that advance our understanding of human behavior. He was also awarded an NIH NIBIB Trailblazer R21 grant for 3 years to study health risk behaviors that involve hand-to-mouth gestures including smoking and overeating. He is currently directing the NIH-funded SenseWhy study, which aims to lay the foundation for studying overeating behaviors among participants with obesity through passive wearable sensors. This study has led to his obtaining an R03 and recently an R01 to validate novel wearable technology for diet and eating assessment in people with obesity. His research has been honored by the National Institute of Nursing Research (NINR) with an Outstanding Poster Abstract Award, Army SBIR with both Phase-I and Phase-II award, and several ACM and IEEE peer-reviewed conferences and journals with a Distinguished Paper Award at ACM IMWUT, a Best Presentation Award at ACM IMWUT, and a Best Poster Award at ACM IMWUT, Best Paper Awards in IEEE Body Sensor Networks (BSN), EAI BODYNETS, The Obesity Society (TOS), and IEEE PerCom (Samsung Best Paper Award).
Dynamic functional brain network analysis using hidden semi-Markov models | Dr. Heather Shappell
Dynamic functional brain network analysis using hidden semi-Markov models
Speaker: Dr. Heather Shappell, Assistant Professor of Biostatistics and Data Science, Wake Forest University School of Medicine
Moderated by Kia Teymourian
April 22, 2021
Abstract: A great deal of evidence now supports the theory that the brain is a system of interacting regions that produce complex behaviors. Functional magnetic resonance imaging (fMRI) data can be used to measure whole brain activity and extract information on which regions or components of the brain interact/communicate (i.e., are functionally connected). While much of the previous brain network literature is based on one average network constructed using data from the entire fMRI scan (i.e., static connectivity), emerging evidence suggests brain network topology exhibits meaningful variations within an fMRI experiment (i.e., dynamic connectivity). I propose a hidden semi-Markov model (HSMM) approach for inferring functional brain networks from fMRI data. Specifically, I propose using HSMMs to find each subject’s most probable series of network states during the course of a scan, the cumulative time spent in each state, and the probabilities of transitioning from one state to another. I will discuss findings from a study where we applied this analysis approach on fMRI data from a cohort of children with Attention-Deficit/Hyperactivity Disorder (ADHD). Finally, I will conclude with a discussion of future research questions and directions.
Speaker Bio: Heather Shappell is currently an Assistant Professor of Biostatistics and Data Science at Wake Forest University. She completed a postdoctoral fellowship in July of 2020 in the Department of Biostatistics at Johns Hopkins University, Bloomberg School of Public Health, where she was awarded a Provost Fellowship to continue her research efforts developing statistical methods to analyze fMRI data. She earned her PhD in Biostatistics from Boston University in 2017, as well as her Masters in Biostatistics from Boston University in 2013. Heather obtained her Bachelor’s degree in Mathematics and Computer Science from Arcadia University in 2011. Her current research interests include the statistical analysis of network data, with a particular focus on applications to neuroscience and brain networks. She has also been involved in the statistical analyses for several clinical trials, including clinical trials for treatment of the rare disease, Progeria, as well as in the analyses for observational studies on mental illness.
The Smart Hospital Living Lab | Dr. Vasillis Kostakos
The Smart Hospital Living Lab
Speaker: Vasillis Kostakos, Professor of Human Computer Interaction, University of Melbourne
Moderated by Professor Reza Rawassizadeh
March 25, 2021
Abstract: In this talk I will present a range of projects we have recently begun under the “Smart Hospital Living Lab” in Melbourne. The work is still ongoing, so I will take this opportunity to received feedback from the audience regarding the direction of our work and our future plans. In this presentation I will describe how we have approached our collaboration with hospitals in Melbourne, and will discuss how we use our expertise in Ubiquitous Computing and user-centered design to solve problems that the hospitals have. The presentation will give a summary of how a living lab approach to research works, and how it can be used to identify problems and solutions by involving multiple stakeholders in the process. The ongoing projects I will present include patient & staff tracking, the smart pillbox, the IV detection project, and handwashing detection.
Speaker Bio: Prof. Vassilis Kostakos is Professor of Human Computer Interaction in the School of Computing and Information Systems at the University of Melbourne. He was previously a Professor at the University of Oulu (Finland). He earned his B.Sc. and Ph.D. in Computer Science from the University of Bath, UK. He is a computer scientist who works in the fields of human-computer interaction and Ubiquitous Computing. His research focuses on how to model user behaviour based on sensor data, and how to develop technologies that better understand and better respond to humans. His work combines user-centered design, machine learning, and mobile & smartphone-based technologies. He has previously led projects on pedestrian mobility at a city-scale, smartphone software for sensing users’ lifestyles, and large-scale analysis of social media. His main expertise is in smartphone sensing, context-aware computing, and behaviour modelling.
Striim, A Next Generation Distributed Streaming Platform | Alok Pareek
Striim, A Next Generation Distributed Streaming Platform
Speaker: Alok Pareek
February 25, 2021
Moderated by Professor Kia Teymourian
Abstract: Striim is a novel end-to-end distributed streaming ETL and intelligence platform that enables rapid development and deployment of streaming applications. A key area of innovation is to process database events in a continuous streaming manner while accommodating for schema drift, and failures. The real-time ETL engine has been architected from ground-up to enable simple, distributed, stateful management of events with E1P semantics. This talk will cover core architectural choices of the real time ETL engine (i) built-in adapters, and caching structures to extract and load data in real-time from legacy and new cloud endpoints (ii) an extensible SQL-based transformation engine to transform events; users can inject custom logic via a component called Open Processor (iv) New primitives like MODIFY, BEFORE and AFTER to manage database change records and (v) built-in data validation that continuously checks if everything is continually making it to the destination. We will cover real world implementation challenges and future open problems.
Guest Speaker Bio: Alok Pareek is Founder and head of engineering at Striim, a Palo Alto based software startup. Alok started his career as a developer in the Oracle kernel development team where he contributed to core redo generation algorithms, point in time media recovery, and high-speed data movement algorithms for ten years. Subsequently, Alok served as CTO and software architect at GoldenGate software, the industry leader in heterogeneous database replication. GoldenGate was acquired by Oracle in 2009. Post-acquisition, Alok led the product strategy for Oracle’s data integration software product portfolio including GoldenGate. Alok also led the engineering and performance teams that collaborated with strategic customers on architecture, and real-world implementations. He holds multiple patents in data management and has presented at numerous academic and industry conferences. Alok holds a graduate degree in Computer Science from Stanford University.
Application of Machine Learning to Construct Investment Portfolios | Dr. Eugene Pinsky
Application of Machine Learning to Construct Investment Portfolios
Speaker: Dr. Eugene Pinsky, Associate Professor of the Practice
Moderated by: Kia Teymourian, Assistant Professor of Computer Science
January 28, 2021
Abstract: One of the primary applications of machine learning methods is to uncover patterns from historical relationships and trends in the data. In unsupervised learning, we apply these methods and let the system itself find structure in the data. The most widely used algorithm in such learning is kmeans clustering that looks for patterns in data and partitions the data points into k groups (“clusters”). The key to using such a method is the existence of similarity (distance) measure with resulting clusters containing “close” points. With clustering, we discover patterns in data and classify data according to cluster membership. This provides a way to describe even the large data sets in much simpler terms.
Such clustering methods have been successfully used in a variety of areas. In many applications, data points could have many dimensions and evolve over time. It is of interest, therefore, not only to identify the clusters but to examine the evolution of the data patterns in time. To address this problem, we divide time into periods, add time periods as an extra description to data points and apply k-means clustering to the resulting data. With this extension, the data patterns within the same time periods are reflected in the same cluster membership, whereas temporal evolution of these patterns can be described by paths in the appropriate (cluster, time) space. As a result, the time behavior of points in a large data set can be described in a much simpler way by simply specifying a trajectory of clusters that these points occupy in the successive time periods. To measure the difference of patterns in time, we propose to use the Hamming distance of their trajectories. This measure is easy to compute and visualize. It has a simple analytical interpretation as the duration of time when data points exhibit different patterns (different clusters).
We illustrate this approach by considering an important practical problem in financial industry: choosing mutual funds to construct diversified investment portfolios. The traditional approach of constructing such portfolios involves computing the correlations between the component funds. However, these correlations change over time, especially during significant market events. This makes it difficult to construct such portfolios in practice. We use historical daily data on thousands of mutual funds over multi-year period. We then apply k-means clustering to the vectors of daily returns for each fund and year. This allows to represent annual correlation patterns of funds by clusters and to describe changing fund correlations by cluster paths. We use Hamming distance of cluster trajectories as a measure portfolio diversification. It has a simple intuitive interpretation as the average time when funds exhibit different performance return patterns. We present some examples and compare the portfolios computed by traditional approach and by clustering.
The proposed method can be used in many applications where we need to analyze and visualize evolution of large multi-dimensional data patterns over time.
Delete: The Forgotten Operator | Dr. Mathos Athanassoulis
Delete: The Forgotten Operator
Speaker: Dr. Mathos Athanassoulis, Assistant Professor
Moderated by: Kia Teymourian, Assistant Professor of Computer Science
December 11, 2020
Abstract: When building new data systems, and their underlying storage managers, typically we optimize performance (access time or throughput), however, as data management tasks are increasingly moving to the cloud and refer to massive data sets a set of other metrics are being considered, including the cost (space amplification, monetary, energy) and the deployment time. In this work we focus on deletion, and we discuss that the typical deletion-through-invalidation approach comes at a cost with respect to space amplification and potentially of the privacy of the users. We focus on the concept of out-of-place deletes which are frequently employed by storage engines that use the log-structure merge (LSM) tree as their backend.
LSM-Trees support high ingestion rates with low read/write interference. These benefits, however, come at the cost of treating deletes as a second-class citizen. A delete inserts a tombstone that invalidates older instances of the deleted key. State-of-the-art LSM engines do not provide guarantees as to how fast a tombstone will propagate to persist the deletion. Further, LSM engines only support deletion on the sort key. To delete on another attribute (e.g., timestamp), the entire tree is read and re-written. In this talk we present how LSM-Trees work and present a family of new techniques that allow for timely deletion without compromising privacy or space amplification, leading in small read performance benefits and near-zero increase in the amortized write amplification.
Speaker Bio: Manos Athanassoulis is an Assistant Professor of Computer Science at Boston University, Director and Founder of the BU Data-intensive Systems and Computing Laboratory and co-director of the BU Massive Data Algorithms and Systems Group. His research is in the area of data management focusing on building data systems that efficiently exploit modern hardware (computing units, storage, and memories), are deployed in the cloud, and can adapt to the workload both at setup time and, dynamically, at runtime. Before joining Boston University, Manos was a postdoctoral researcher at Harvard School of Engineering and Applied Sciences. Manos obtained his PhD from EPFL, Switzerland, and spent one summer at IBM Research, Watson. Manos’ work is published on top conferences and journals of the community, like ACM SIGMOD, PVLDB, ACM TODS, VLDBJ, and others, and has been recognized by awards like “Best of SIGMOD” in 2016, “Best of VLDB” in 2010 and 2017, and “Most Reproducible Paper” at SIGMOD in 2017. Manos has been acting as a program committee member and technical reviewer in top data management conferences and journals for the past ten years and is chairing ACM SIGMOD Reproducibility as of Fall 2020.