Q&A: What Will BU’s Data Science Classroom Experience, the Curriculum, Look Like?
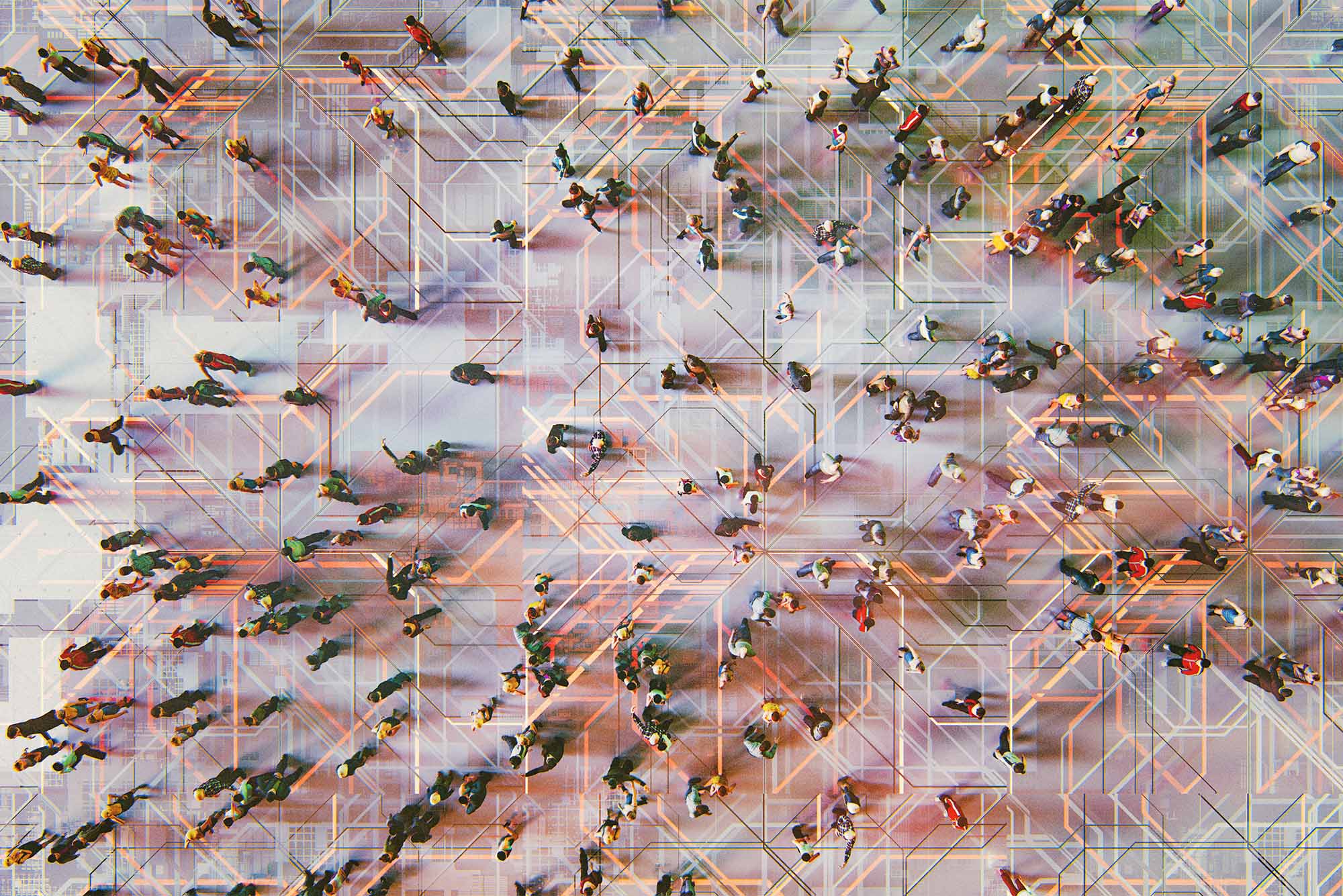
Photo by Getty Images/Gremlin
Q&A: What Will BU’s Data Science Classroom Experience, the Curriculum, Look Like?
“It’s less about the skills and more about a way of thinking that lasts a lifetime,” says Azer Bestavros
In a wide-ranging and detailed conversation, Azer Bestavros, BU’s inaugural associate provost for computing and data sciences and a William Fairfield Warren Distinguished Professor, outlines what undergraduate and PhD students will see under a new faculty in a new program
Q&A
With Azer Bestavros
BU Today: You’ve talked about how the computing and data sciences curriculum at BU differs from others. Can you talk about what in your view makes it unique?
Bestavros: The way I like to describe it is that for a typical discipline, you’re preparing students to become professionals in a particular field. So if you are interested in working in robotics as a field, you go study mechanical engineering. That’s the discipline. Your education in computing and data sciences at BU is different, because you can think of it as a foundation for pretty much every possible career. To that end, our curriculum takes an alternative approach—first you build a core in data science, and then you actually steer your way into whatever field you want to focus on. As a result, there will be a variety of combinations of disciplines that go into our data science education.
Think of it as a platform on which you add other things. This is where you align your passion with your career. So if my passion is the environment, I need to connect knowledge about maybe earth and environment or environmental sciences or sustainability with the competencies and skills needed for a long career, right? But you first need the foundation to go forward. Data science gives you that foundation. And then you build on it.
BU Today: What you’re describing sounds a lot like liberal arts, a foundational education people use to branch off into hundreds of different fields. It’s been said that data science is the liberal arts of this generation. Do you think that’s accurate?
Bestavros: I think it’s very accurate. Data science is critical thinking, it’s quantitative reasoning, it’s communication. It’s learning how to continue to learn throughout your life. It’s less about the skills and more about a way of thinking that lasts a lifetime. And it’s very common across disciplines.
BU Today: If I am a student who leans toward liberal arts and away from math and science, how will the CDS curriculum help me?
Bestavros: Well, there’s certainly a math component to data science. But if you want to have an impact in a world that is going to be 100 percent data-driven, this will absolutely be of help to you, whether you think of politics, you think of communication, you think of business, you think of marketing, you think of public health, you think of social work. Going back to what we talked about as far as liberal arts, well, we have to learn how to critically think and express our thoughts in writing, of course. But now we all need to know how to do that with numbers, because that’s the language now that one has to use.
BU Today: So how will the language of numbers help someone pursuing a more liberal arts background?
Bestavros: Well, for starters, critical thinking. I have to be able to look at not just numbers, but what those numbers mean. This is going to be baked into everything we work with. And if you want to think critically and to leverage the tools of data science, you have to learn how to use them, not in order to develop new technologies, but in order to solve problems, wherever your passion lies.
So for example, if your passion is history, there is now a lot of interest in looking at historic artifacts through the lens of data. Think of artifacts as numbers, think of maps and charts as geographic information systems that you can interrogate to understand or interpret history. Without data science, you are unable to converse at the right level for many of these disciplines.
BU Today: How about a specific course? Can you give examples of new CDS courses that might cross into several disciplines?
Bestavros: So we thought a lot about this. So one course, the first course, if you have not touched anything else to do with data science, is called DS-100: Data Speak Louder Than Words. Another description of it is a day in the life of, or a semester in the life of, a data scientist. So you’re going to spend one semester taking this class, and you will know something about what data science is about. You get a taste of the kind of things that a data scientist has to work with, from getting real data, cleaning the data, starting to make some hypotheses about something you want to look into with the data, testing these hypotheses to come out with some conclusions, and eventually visualizing and communicating these to a public audience.
In one course, you get to see all of this. And if that’s the one course you’re going to take in data science, great. You now can go out and say, ‘I know what data science is.’ You’re not an expert, you’re not yet there. But at least you get a flavor of it.
Now, beyond that, we have sequences of courses that adopt the same approach, where you learn the math, and you learn the programming that you need to solve a problem. We decided to go away from the typical gateway courses in other disciplines to on-ramp courses. Rather than make students wait until they are done with a whole bunch of prerequisites to take a course, say, in machine learning, we actually introduce machine learning in the very first class. It’s very elementary, but you get to taste what machine learning is like, and later on you get to deepen your understanding as you build the necessary competencies.
Here’s another example. After DS-100, the next three classes are mathematically oriented classes called Foundations of Data Science I, II, and III. Every one of them is iterating through various mathematical concepts and algorithms by showing how they can be put into programs to solve real-world applications. So instead of thinking about Foundations of Data Science as silos of courses that act as prerequisites to future learning, we actually start from the get-go, putting your hands on real data, teaching you the math and the programming that you need when you need it.
BU Today: You mentioned a phrase I want to go back to—problem solving. Is this is one of the core principles that make data science so important to the world—it helps solve problems?
Bestavros: Yes, that’s true. But I would go a step further. It helps with understanding the problem first, and then solving it. I’ll give you an example. Let’s take something in the social sciences, like getting visibility into political engagement and participation, or who votes for what. Well, we know there’s data out there. Can I take this data and get a picture out of it that may actually indicate that there is a question to ask or a problem to solve?
Problem solving presumes that somebody figured out what the problem is already. But actually figuring out the problem is itself the first step. In a world where everything is reflected in the data, you’re not going to find a problem unless you actually look at the data to make the problem crystal clear.
Why do people refuse to get vaccinated? In the old world, I just come up with a hypothesis, I could write an essay. Well, why not let the data speak? What does the data tell you about what people are doing? What kind of data collection can I do to help me figure out these things? Where can I find this data?
BU Today: And, to take that one step further, once you identify the problem. . .
Bestavros: There is problem solving.
The reason I’m saying this is to give more credit to the science in data science. In disciplines like engineering, it really is about solving well-defined problems. Here’s the client, they tell you what they want, and you will develop the solution for them. But data scientists are themselves the ones working with experts to figure out what is the right question to even ask. This underscores the notion of data science being like liberal arts. It really is about first understanding the world. And then solving the problem.
BU Today: Is there anything else about the curriculum you think is important to talk about?
Bestavros: It’s all about impact and empowerment. This is a curriculum designed to make you feel, ‘I can go start a company if I need to.’ Or become an activist to do whatever you want.
BU Today: But anybody can be an activist, right? Anybody can have a strong opinion, attend protests, and launch a fundraising effort for a cause. How does data science help someone become a better activist?
Bestavros: Evidence-based policymaking is a big word nowadays. When people think about pushing new solutions or advocating for change, it’s important to ask: what’s your evidence for that? Data science is giving you the power to do what you want to do. This goes back to what we discussed earlier; it’s really about aligning your passion with a career. If you want to be an effective marketing person, no matter where you go, someone is going to say: what’s your evidence why this idea should work?
BU Today: We’ve talked largely about the undergraduate experience. What about PhD students?
Bestavros: Very important. Let’s say you have a faculty member on the Medical Campus working on cancer research, and they want to work with a PhD student, using machine learning. It’s actually very hard because that PhD student who knows how to develop or adapt machine learning for cancer research is probably in computer science, admitted by a computer science faculty member, and largely invisible to faculty elsewhere.
Our program is created to allow for a more porous boundary, so they can go out to sit in somebody’s lab and see what it means to do machine learning in the context of medical research, or in the context of neuroscience, chemistry, or physics. The PhD program is for students who actually want to do their degrees, their scholarship, in things like data science and machine learning and artificial intelligence (AI), but they don’t want to do it in the context of computer science. They want it in the context of the environment, or medicine, or communication, or whatever.
BU Today: Finally, aside from the classroom learning experience, how will CDS incorporate outside, real-world experiences into the curriculum?
Bestavros: That’s the experiential learning component of our curriculum. The only way to learn the power of something like data science is to see it in action, not just hypothetical, or classroom academics, but things that connect with what we do, day in and day out. We like to say experiential learning is important early and often. Don’t wait until your senior year to do your practicum, you can actually engage in experiential learning as a sophomore, you can even do it as a freshman.
To get us there, we are leveraging the incredibly successful BU Spark! programs, which are now offered through CDS. We are also extending it by offering one-credit or two-credit workshops very early on, to start introducing you to tools that you may not see in a class, but are actually very crucial if, for example, you want to go and get US Census data. If you’re going to start thinking about doing a project that looks at data from open sources, like the Census, how do you do that? What’s the UX, what’s the user experience? There is a workshop just on that.
Think of these workshops like taking a course in dancing. It’s credit. But it adds to your skill set, something that will make you ready to do an internship or to take on a real project from the City of Boston, or some nonprofit, and to work on it as part of either curricular or extracurricular work within CDS. We thought a lot about experiential learning. And it’s really baked into everything we do. This also helps students realize that they don’t have to wait to be a senior to start seeing the impact of what they do.
Comments & Discussion
Boston University moderates comments to facilitate an informed, substantive, civil conversation. Abusive, profane, self-promotional, misleading, incoherent or off-topic comments will be rejected. Moderators are staffed during regular business hours (EST) and can only accept comments written in English. Statistics or facts must include a citation or a link to the citation.