An Introduction to Machine Learning for Speech-Language Pathologists: Concepts, Terminology, and Emerging Applications
Cordella, C., Marte, M. J., Liu, H., & Kiran, S. (2024). An Introduction to Machine Learning for Speech-Language Pathologists: Concepts, Terminology, and Emerging Applications. Perspectives of the ASHA Special Interest Groups. https://doi.org/10.1044/2024_PERSP-24-00037
Read Paper
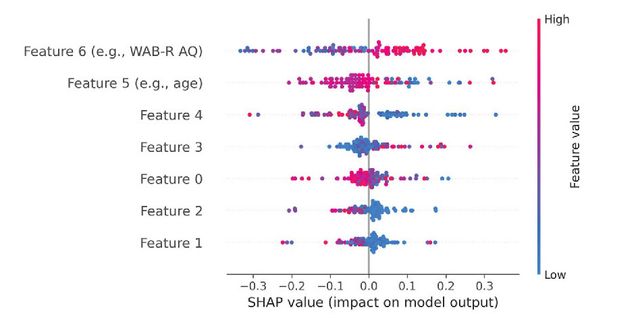
Purpose
The purpose of this article is to orient clinicians and researchers to machine learning (ML) approaches, as applied to the field of speech-language pathology. We first introduce key ML concepts and terminology and proceed to feature exemplar papers of recent work utilizing ML techniques in speech- language pathology. We also discuss the limitations, cautions, and challenges to the implementation of ML and related techniques in speech-language pathology.
Q&A with Manny Marte
What is this paper about and why are the results important?
This paper provides an introductory overview of machine learning (ML) concepts, applications, and challenges in speech-language pathology, with a focus on aphasia research, to help clinicians and researchers navigate this emerging technology’s potential impacts on diagnosis, assessment, and treatment planning. We review exemplar studies demonstrating how ML approaches can enhance diagnostic accuracy, severity staging, and outcome prediction in aphasia above and beyond traditional methods. We also emphasize the need for clinically interpretable and validated models, highlighting both the promise and current limitations of ML in speech-language pathology practice.
How do the findings relate to the brain and recovery?
The paper’s application to brain recovery is demonstrated in its review of how machine learning (ML) can enhance diagnosis, prognosis, and personalized treatment planning for disorders like aphasia. The discussed ML models, which integrate multimodal data, including neuroimaging, have the potential to provide deeper insights into recovery mechanisms and identify key factors influencing recovery outcomes.