Development and applications of fragment based drug design methods
Sandor Vajda, Karen Allen, George Keseru, and Adrian Whitty
Supported by:
NIH R35 GM118078 “Computational Mapping of Proteins for the Binding of Ligands” (PI: Vajda)

Fragment-based drug design (FBDD) is a combinatorial approach in which individual fragments binding to regions of the target site are selected from a fragment library, and then combined to form potential lead compounds. Fig. 1 shows a binding site comprised of three pockets. The first step of the procedure is the mapping of the binding site to identify the subsites or pockets and to find molecular fragments that bind in particular pockets with some preference. FBDD also includes design by combining fragment hits around a template (Fig. 1c), or by growing out from an individual fragment (Fig. 1d). These steps of FBDD are beyond the scope of this proposal, but we try to generate reliable information on the subsites of the binding site and on the functional groups that bind at these subsites with some preference.
Fragment based design is based on the observation that the binding sites of proteins generally include smaller regions called hot spots that are major contributors to the binding free energy, and hence are crucial to the binding of any ligand at that particular site. In drug design applications such hot spots can be identified by screening for the binding of fragment-sized organic molecules. Since the binding of the small compounds is very weak, it is usually detected by Nuclear Magnetic Resonance (NMR) or by X-ray crystallography methods. Results confirm that the hot spots of proteins bind a variety of small molecules, and that the fraction of the “probe” molecules binding to a particular site predicts the potential importance of the site and can be considered a measure of druggability.
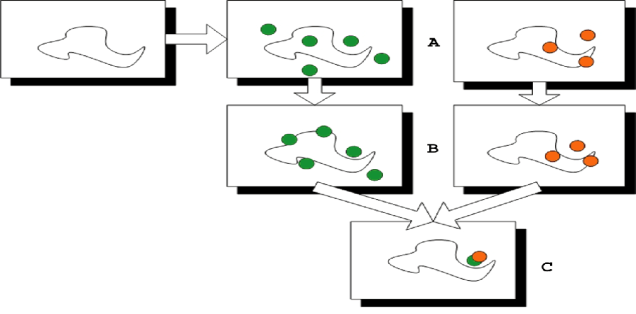
The Vajda lab has developed computational solvent mapping as a computational analogue of the NMR and X-ray based screening experiments. The method places molecular probes – small organic molecules containing various functional groups – on a dense grid defined around the protein, finds favorable positions using empirical free energy functions, further refines the selected poses by free energy minimization, clusters the low energy conformations, and ranks the clusters on the basis of the average energy. To determine the hot spots, we find consensus sites, i.e., regions on the protein where clusters of different probes overlap, and rank these sites in terms of the number of overlapping probe clusters. This principle is illustrated by the schematic figure (Fig. 2) for the case of mapping a protein with only two probes (represented as green and red dots, respectively), each forming a few clusters on the protein surface. While the clusters overlap in the main consensus site, the distributions of different probes may slightly differ, resulting in the arrangement shown in Fig. 2c. Thus, in principle the mapping can identify both the “hot spots” of the binding site and the functional groups that tend to bind at specific locations within it. The consensus site, binding the largest number of probe clusters, is considered the main hot spot. The number of probe clusters at a particular consensus site (CS) correlates with the importance of that site for binding. The main hot spot and other hot spots within a 7 Å radius predict a site that can potentially bind drug-size ligands. The mapping algorithm has been used in a variety of applications, provides insight on molecular recognition, and opens new possibilities for drug design.
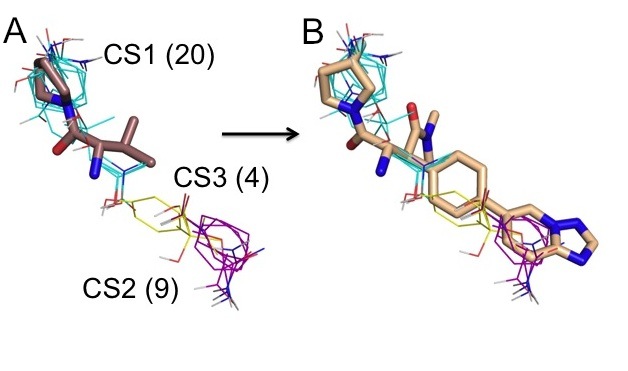
The hot spots obtained by computational mapping identify the regions that are most likely to substantially contribute to the binding free energy and hence are the most important regions for drug design. We have recently shown that the top ranked consensus cluster, predicting the main hot spot, generally coincides with the initial fragment hit, whereas information on the secondary hot spots provides a rational basis for expanding the core fragments into higher affinity lead candidates. Indeed, high-affinity ligands extend beyond the main hot spot into other hot spots, in most cases identified by the second, third, and possibly fourth ranked consensus clusters. As an example, Fig. 3A shows mapping results for the ligand-free Dipeptidyl Peptidase IV (DPPIV, PDB code 1j2e). An initial fragment hit is superimposed to show that it binds to the larges consensus site CS1 with 20 probe clusters. An optimized inhibitor, shown in Fig. 3B, extends into consensus clusters ranked 2 and 3. The secondary hot spots, defined by consensus clusters 2 and 3, contribute to the binding free energy but have weaker interactions with ligands than the main hot spot. Nevertheless, as shown in Fig. 3B, the inhibitor matches the three top ranked consensus clusters very well, and this is likely to contribute to its respectable ligand efficiency and the high affinity (IC50 = 4.3 nM). More generally, the secondary hot spots identified by the computational mapping reveal whether the core fragment can be expanded and, if so, into which directions.
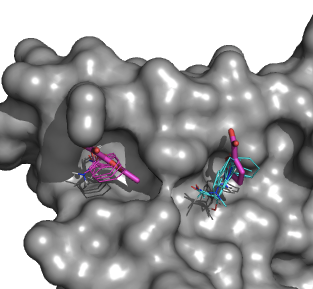
In collaboration with the Allen and Whitty labs we integrate computational and X-ray crystallographic mapping methods by pre-selecting the compounds for the X-ray based screening by computational mapping. The approach is validated by mapping model systems and systems of current pharmaceutical interests. The comparison of predicted and observed interactions helps us to better understand the principles that govern the binding of fragment-sized molecules to functional sites of proteins, and to further improve the mapping. For example, we have expressed human IL-2 in E. coli, purified the protein by refolding from inclusion bodies, and obtained crystals that diffract to 2.3Å resolution. With the additional optimization of kinetic conditions including oil over the reservoir and temperature, we have obtained crystals that diffract to 1.9 Å resolution. This resolution is sufficient to see the binding of small chemical fragments (MW ~200 Da), even considering the potential loss of up to 0.5Å resolution from the high concentration (~ 100 mM) soaks of these fragments. Moreover, this plate crystal form is highly reproducible crystals that were either soaked or co-crystallized with one of the many fragments derived from the known IL-2 inhibitors. Our first success was the crystal soaked with the small fragment 5-methyl-2-furoic acid. The X-ray crystal structure was obtained to 1.9Å resolution. The resulting structure shows excellent agreement between computational and experimental mapping (Fig. 4).
Publications:
Yueh C, Rettenmaier J, Xia B, Hall DR, Alekseenko A, Porter KA, Barkovich K, Keseru G, Whitty A, Wells JA, Vajda S, Kozakov D. Kinase Atlas: Druggability Analysis of Potential Allosteric Sites in Kinases. J Med Chem. 2019 Jul 25;62(14):6512-6524. doi: 10.1021/acs.jmedchem.9b00089.
Egbert M, Whitty A, Keseru GM, Vajda S. Why Some Targets Benefit from Beyond Rule of Five Drugs. J Med Chem. 2019 Jun 26. doi: 10.1021/acs.jmedchem.8b01732.
Wakefield AE, Mason JS, Vajda S, Keseru GM. Analysis of tractable allosteric sites in G protein-coupled receptors. Sci Rep. 2019 Apr 16;9(1):6180. doi: 10.1038/s41598-019-42618-8.
Vajda S, Beglov D, Wakefield AE, Egbert M, Whitty A. Cryptic binding sites on proteins: definition, detection, and druggability. Curr Opin Chem Biol. 2018 Jun;44:1-8. doi: 10.1016/j.cbpa.2018.05.003.
Beglov D, Hall DR, Wakefield AE, Luo L, Allen KN, Kozakov D, Whitty A, Vajda S. Exploring the structural origins of cryptic sites on proteins. Proc Natl Acad Sci U S A. 2018 Apr 10;115(15):E3416-E3425. doi: 10.1073/pnas.1711490115.
Whitty A, Zhong M, Viarengo L, Beglov D, Hall DR, Vajda S. Quantifying the chameleonic properties of macrocycles and other high-molecular-weight drugs. Drug Discov Today. 2016 May;21(5):712-7. doi: 10.1016/j.drudis.2016.02.005.
Hall DR, Kozakov D, Whitty A, Vajda S. Lessons from Hot Spot Analysis for Fragment-Based Drug Discovery. Trends Pharmacol Sci. 2015 Nov;36(11):724-736. doi: 10.1016/j.tips.2015.08.003.
Vajda S, Whitty A, Kozakov D. Fragments and hot spots in drug discovery. Oncotarget. 2015 Aug 7;6(22):18740-1.
Kozakov D, Hall DR, Napoleon RL, Yueh C, Whitty A, Vajda S. New Frontiers in Druggability. Med Chem. 2015 Dec 10;58(23):9063-88. doi: 10.1021/acs.jmedchem.5b00586.
Kozakov D, Hall DR, Jehle S, Luo L, Ochiana SO, Jones EV, Pollastri M, Allen KN, Whitty A, Vajda S. Ligand deconstruction: Why some fragment binding positions are conserved and others are not. Proc Natl Acad Sci U S A. 2015 May 19;112(20):E2585-94. doi: 10.1073/pnas.1501567112.
Zerbe BS, Hall DR, Vajda S, Whitty A, Kozakov D. Relationship between hot spot residues and ligand binding hot spots in protein-protein interfaces. J Chem Inf Model. 2012 Aug 27;52(8):2236-44. doi: 10.1021/ci300175u.