POV: COVID-19 Shows Us We Need Rapid Response Data Science Teams
Eric Kolaczyk, Director of Hariri Institute of Computing and Faculty Affiliate of CISE, contributed an opinion piece published by BU Today on June 4th entitled “POV: COVID-19 Shows Us We Need Rapid Response Data Science Teams.” In it, Professor Kolacyzk describes how the COVID-19 pandemic is not only an indication of the need for development but also a rapid response from data scientists.
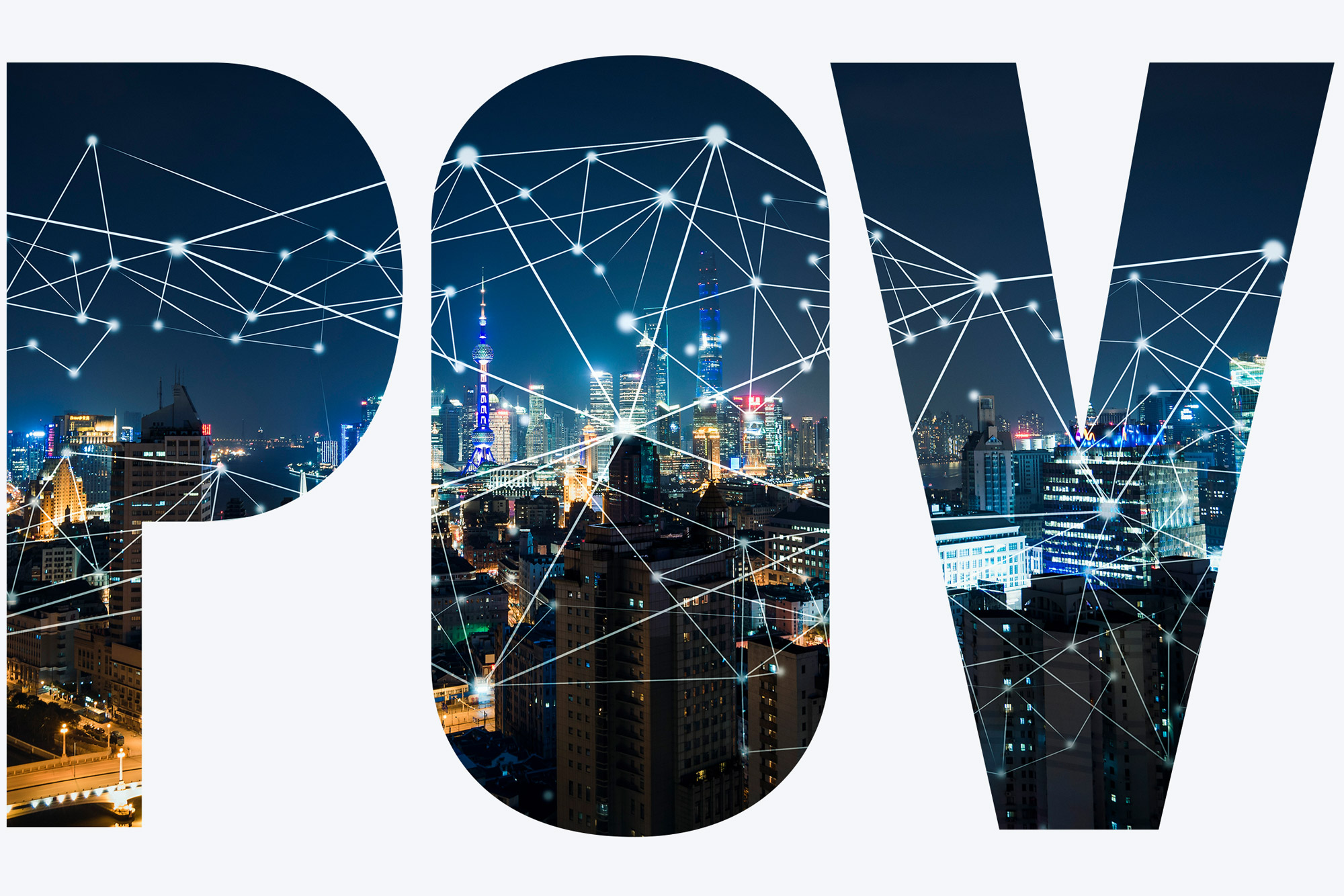
Photo by Shaxiaozi/iStock
During the COVID-19 pandemic, for better and for worse, we are learning much about ourselves as a society. One lesson that’s become especially clear is the critically important role data science can play in times like this.
Simply put, we’re not prepared—despite over a decade of intensive ramp-up in our capacity for data science education, despite the ubiquity of data-driven innovation, business, and decision-making across the world, despite our ability to do incredibly sophisticated artificial intelligence (AI) computation. Somehow, despite all of this, we are still not prepared for the data-centric challenges needed to manage and control this current pandemic.
That “we don’t have all the data” is a complaint we’ve heard far and wide at this point and it’s absolutely true. But it’s an easy excuse. More fundamentally, the point is that we’re not prepared to collect the data. We’ve struggled to track who is infected and when, where they got infected and how, whether or not they’ve been tested and when they’ve recovered, and ultimately, who they might have infected in turn.
Moreover, just as importantly, even if we had all of that data—collected by a multitude of cities, states, and countries, schools and businesses, airports and train stations, and whatnot—we’re not uniformly prepared to wrangle the data into a usable form, to analyze the data, and extract empirically grounded and actionable insights from the data to help inform decision-making and policies. That is, we’re not prepared to do data science as we’d like to and should.
As far back as 1854, during London’s cholera epidemic, John Snow famously demonstrated the profound impact that data science (done well) can have in controlling infectious disease, when he used a combination of hospital and public records, along with interviews, to produce a map of cases that implicated the now-infamous Broad Street pump as the local source of the epidemic. The result of Snow’s data science exercise is said to have involved removing the handle of the pump, and thus preventing residents from drawing tainted water.
If only the solution were as simple for the coronavirus. Unfortunately, the scale of today’s pandemic is vastly greater than that of the London cholera epidemic. In turn, so too is the scale of the data science challenges we face.
None of this is to dismiss the enormous collective data science efforts across the country, and the world more generally, that have been critical in supporting and informing what success we have had in our management and control of COVID-19. But what’s missing from this pandemic—this global emergency—is a level of coordination and integration among these efforts that emergency responders, militaries, and related units around the world have found is vital to optimizing their effectiveness.
True, the data science community has responded rapidly with all that we have done. And, at the risk of overusing the phrase, perhaps even heroically in many ways, large and small. Nevertheless, we have not produced a rapid response for a crisis that has seen hundreds or thousands of people dying every day around the world for more than three months.
We haven’t had a pandemic for 100 years. And data science, at least in its modern form, is an area that is still quite young. So it is understandable, and perhaps even to be expected, that we find ourselves insufficiently prepared for the current crisis we face.
What’s missing is the shared infrastructure, the planning, and the preparedness training. What’s missing is a notion of units or teams that are agile yet interoperable, working with a shared set of standards and tools, with a clear and efficient set of communication channels. What’s missing are data science rapid response teams.
Without an established network of data science rapid response teams, there is a tremendous inefficiency to our current efforts. Governments at local, regional, and national levels, as well as academic institutions, large businesses, hospitals, and the like, are right now all going through basically the same data-centric exercises everywhere—looking to quickly find and tap the right data science expertise and set up infrastructure around data collection and monitoring, contact tracing, epidemiological modeling, reporting, and planning. We’re all inventing the proverbial wheel at the same time. But the inherent inefficiency is costing human lives and livelihoods.
When you add to this chaos the impact of disparities that we’re beginning to witness across different communities relative to both the susceptibility to poorer COVID-19 outcomes and a reduced ability to respond effectively—disparities highly correlated with existing disparities along race, gender, and socioeconomic dimensions—the need for a different approach is painfully clear.
We haven’t had a pandemic for 100 years. And data science, at least in its modern form, is an area that is still quite young. So it is understandable, and perhaps even to be expected, that we find ourselves insufficiently prepared for the current crisis we face. Nevertheless, we cannot afford to wait until the next pandemic—nor even the next wave of the current one—to change the situation. We need to act now to begin developing a national, and ideally, global, network of data science rapid response teams.
To some extent, many of the necessary raw materials for such a rapid response network in data science are already available. There are thousands of data scientists embedded in local efforts around the country, and the world, contributing towards monitoring and control of COVID-19. Most already are contributing through self-organized units (such as universities) and even, to a lesser extent, through various emerging loose collections of units (independent consortia, governmental task forces, and so on). Their resulting efforts have demonstrated a capacity to make large amounts of specialized knowledge available in a very short period of time to the researchers working tirelessly to develop a vaccine.
They’ve demonstrated progress towards previously unheard of levels of openness around responsible and equitable data sharing. And they’ve demonstrated unprecedented levels of collaboration around development of algorithms and software, such as for statistical modeling and prediction or for privacy-preserving automated contact tracing via cell phones.
What’s missing is the shared infrastructure, the planning, and the preparedness training. What’s missing is a notion of units or teams that are agile yet interoperable, working with a shared set of standards and tools, with a clear and efficient set of communication channels.
But a true rapid response network for data science is unlikely to emerge fully and well-formed on its own. The tent that is “data science” sits over the intersection of a spectrum of what has traditionally been different communities of scientific expertise. An intersection that is still in the early stages of developing a mature sense of itself as a community. At the same time, much of the culture and spirit of data science has been one of innovation, exploration, disruption, and change. Which has proven to be incredibly conducive toward progress in a host of computing-enabled and data-driven areas that have profoundly transformed us and our society. But the emergency around us is pushing the data science community to do something different. To work differently. And while that community has clearly demonstrated a willingness and capacity to do so, a critical level of organizational leadership and direction is needed to take it to the next level.
Certainly some aspects of the challenge we face are due to its sheer scale. And there are still technical challenges to be solved and deployed, such as where data and model sharing meet questions of ethics and privacy. But beyond the scale and the technology, we must begin to think differently, in terms of how best to structure, equip, and prepare our data science assets—human and otherwise—to be brought to bear most effectively and equitably in emergency situations. An element of structure and training is needed, in coordination with local, national, and ideally, even global government, perhaps in the spirit of the National Guard. An element of organized and persistent volunteerism is needed, as well as a willingness to “go” wherever and whenever the need arises. In short, we have to be nimble like a speed boat, and not hulking like an ocean liner.
Is the middle of a pandemic the right time to start working on all of this? Yes, absolutely. In doing so, we can both leverage and better channel the tremendous resources we’ve already invested in, as well as the energy and synergy that has resulted around data science, in response to COVID-19. And when the next national or global emergency comes around—whether driven by disease, accidents, or natural disaster—we can know that we’re prepared to deliver a data-driven rapid response. Rather than simply responding rapidly.
“POV” is an opinion page that provides timely commentaries from students, faculty, and staff on a variety of issues: on-campus, local, state, national, or international. Anyone interested in submitting a piece, which should be about 700 words long, should contact John O’Rourke at orourkej@bu.edu. BU Today reserves the right to reject or edit submissions. The views expressed are solely those of the author and are not intended to represent the views of Boston University.